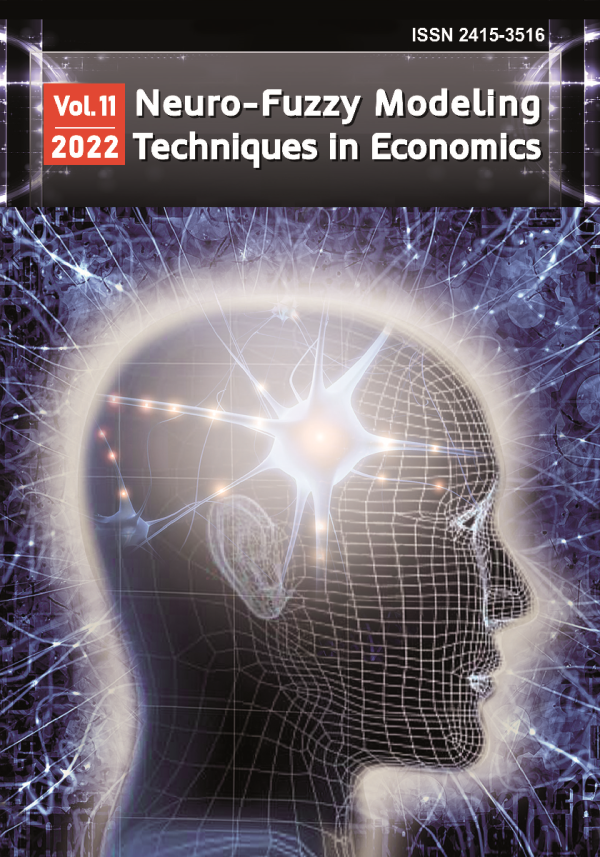
Neuro-Fuzzy Modeling Techniques in Economics
ISSN 2415-3516
Біннінг у нейромережевих скорингових моделях
Binning in neural network scoring models
DOI:
10.33111/nfmte.2016.060
Анотація: Стаття присвячена розробці методологічного підходу до категоризації вхідних показників економіко-математичних моделей оцінювання кредитоспроможності позичальників комерційних банків. Основою математичного інструментарію обрано нейронну мережу типу багатошаровий персептрон. Об’єктом дослідження є процес категоризації пояснюючих змінних скорингових моделей. Предметом дослідження є сукупність методів категоризації та способів оцінки їх впливу на точність моделі оцінювання ймовірності невиконання умов договору позичальником. У результаті проведених експериментальних досліджень у рамках запропонованого методологічного підходу було обґрунтовано здійснювати оптимізацію розбиття на категорії вхідних змінних моделі за рахунок максимізації значення коефіцієнта Джині як показника адекватності скорингових моделей. Було отримано висновок, що зниження показника інформаційної значущості не завжди виступає індикатором погіршення якості класифікатора.
У статті також був розширений список рекомендацій щодо проведення біннінгу, який може бути використаний для побудови більш точних моделей оцінювання кредитоспроможності позичальників комерційних банків
Abstract: The article is devoted to developing methodological approach of categorizing the input variables of economic and mathematical models of evaluating of creditworthiness of commercial banks’ borrowers. The basis of mathematical tools was chosen neural network of type of multilayer perceptron. The object of study is the process of categorizing explanatory variables of scoring models. The subject of study is a set of methods of categorizing and approaches to evaluate their impact on the efficiency of the model of estimation of probability of borrower’s default under the contract. As a result of experimental studies within the confines of the proposed methodological approach it was decided to optimize the input variables partitioning into categories by maximizing the Gini coefficient as a measure of the adequacy of scoring models. A conclusion that a drop in the information value does not always act as an indicator of the deterioration of the classifier was obtained.
In this article it has been expanded a list of recommendations for binning that may be used to build more accurate models of evaluating the creditworthiness of borrowers of commercial banks.
Ключові слова: вагомість ознаки (WOE), інформаційна значущість (IV), біннінг, скорингова модель, нейронна мережа
Key words: weight of evidence (WOE), information value (IV), binning, scoring model, neural network
УДК: 004.622:519.86
UDC: 004.622:519.86
JEL: 52 99 C13 C45 G21
To cite paper
In APA style
Kolyada, Y., & Bondar, V. (2016). Binning in neural network scoring models. Neuro-Fuzzy Modeling Techniques in Economics, 5, 60-80. http://doi.org/10.33111/nfmte.2016.060
In MON style
Коляда Ю.В., Бондар В. Біннінг у нейромережевих скорингових моделях. Нейро-нечіткі технології моделювання в економіці. 2016. № 5. С. 60-80. http://doi.org/10.33111/nfmte.2016.060 (дата звернення: 06.07.2025).
With transliteration
Kolyada, Y., Bondar, V. (2016) Binninh u neiromerezhevykh skorynhovykh modeliakh [Binning in neural network scoring models]. Neuro-Fuzzy Modeling Techniques in Economics, no. 5. pp. 60-80. http://doi.org/10.33111/nfmte.2016.060 [in Ukrainian] (accessed 06 Jul 2025).
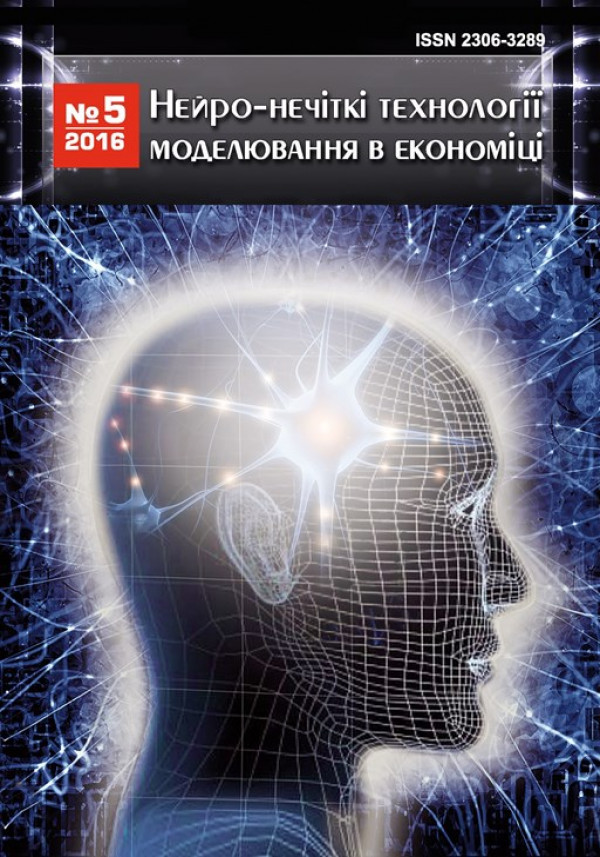
Download Paper
310
Views
72
Downloads
0
Cited by