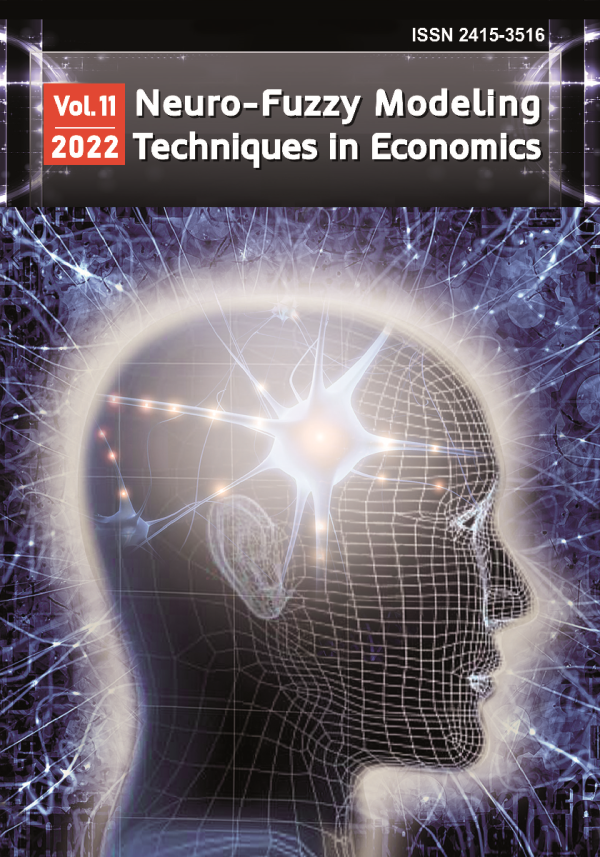
Neuro-Fuzzy Modeling Techniques in Economics
ISSN 2415-3516
Застосування методів машинного навчання до прогнозування часових рядів криптовалют
Machine learning approach for forecasting cryptocurrencies time series
DOI:
10.33111/nfmte.2019.065
Анотація: Робота присвячена питанням прогнозування короткострокової динаміки часових рядів криптовалют за допомогою методів машинного навчання (ML). Проаналізовано методологічні засади, переваги та недоліки використання ML-алгоритмів при дослідженні фінансових часових рядів.
Проведено прогнозні розрахунки динаміки трьох найбільш капіталізованих криптовалют (Bitcoin, Ethereum, Ripple) на 90-денний часовий горизонт як за допомогою запропонованого методу бінарного авторегресійного дерева (BART), так і з використанням нейронних мереж (багатошарового персептрону, MLP) та ансамблю моделей дерев регресії та класифікації (випадковий ліс, RF). Перевагою побудованих моделей є те, що їх застосування не накладає жорстких обмежень на статистичні властивості досліджуваних часових рядів, при цьому в якості предикторів використовуються лише минулі значення цільової змінної.
Проведено порівняльний аналіз прогностичних властивостей побудованих моделей, згідно з яким усі моделі в цілому достатньо адекватно описують динаміку досліджуваних криптовалют, при цьому похибки прогнозу цін за середньою абсолютною процентною похибкою (MAPE) для моделей BART та MLP склали в середньому 3,5 %, а для моделі RF — в межах 5 %.
Проведені комп’ютерні експерименти підтвердили доцільність застосування розглянутих ML моделей для задач короткострокового прогнозування фінансових часових рядів. Побудовані моделі та їх ансамблі можуть бути покладені в основу алгоритмів для автоматизованих торгових систем, призначених для інтернет-трейдінгу.
Abstract: This paper is devoted to the problems of the short-term forecasting cryptocurrencies time series using machine learning approach. The methodological principles, advantages and disadvantages of using ML-algorithms in the study of financial time series are analyzed.
The 90-day time horizon of the three most capitalized crypto-currencies (Bitcoin, Ethereum, Ripple) dynamics has been estimated both using the proposed Binary Autoregressive Tree model (BART), and Neural Networks (Multilayer Perceptron, MLP) and an ensemble of Classification and Regression Trees models (Random Forest, RF). The built models have such advantages as their application does not impose strict limitation on the statistical properties of the studied time series, with only past values of the target variable being used as predictors.
Comparative performance of the predictive ability of the constructed models is carried out, according to which all the models adequately describe the dynamics of the cryptocurrencies. Price forecast errors calculated by the MAPE for the BART and MLP models were on average 3.5 %, and for RF model — within 5 %.
Computer experiments have confirmed the feasibility of using proposed ML-models for the short-term forecasting of financial time series. The constructed models and their ensembles can become the basis for the algorithms for automated trading systems for online trading.
Ключові слова: машинне навчання, модель бінарного авторегресійного дерева, випадковий ліс, нейронна мережа, короткостроко-ве прогнозування, криптовалюта, фінансовий часовий ряд
Key words: machine learning, binary autoregressive tree model, regression and classification tree ensemble, neural network, short-term forecasting, cryptocurrency, financial time series.
УДК: 519.868:339.92
UDC: 519.868:339.92
JEL: C53 C63 G10
To cite paper
In APA style
Derbentsev, V., Velykoivanenko, H., & Datsenko, N. (2019). Machine learning approach for forecasting cryptocurrencies time series. Neuro-Fuzzy Modeling Techniques in Economics, 8, 65-93. http://doi.org/10.33111/nfmte.2019.065
In MON style
Дербенцев В., Великоіваненко Г.І., Даценко Н. Застосування методів машинного навчання до прогнозування часових рядів криптовалют. Нейро-нечіткі технології моделювання в економіці. 2019. № 8. С. 65-93. http://doi.org/10.33111/nfmte.2019.065 (дата звернення: 27.07.2024).
With transliteration
Derbentsev, V., Velykoivanenko, H., Datsenko, N. (2019) Zastosuvannia metodiv mashynnoho navchannia do prohnozuvannia chasovykh riadiv kryptovaliut [Machine learning approach for forecasting cryptocurrencies time series]. Neuro-Fuzzy Modeling Techniques in Economics, no. 8. pp. 65-93. http://doi.org/10.33111/nfmte.2019.065 [in Ukrainian] (accessed 27 Jul 2024).
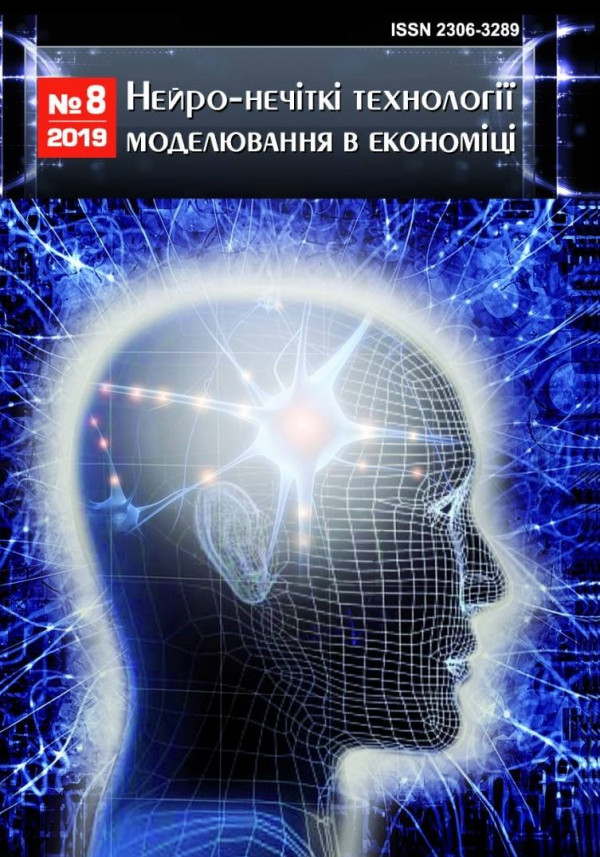
Download Paper
246
Views
54
Downloads
2
Cited by