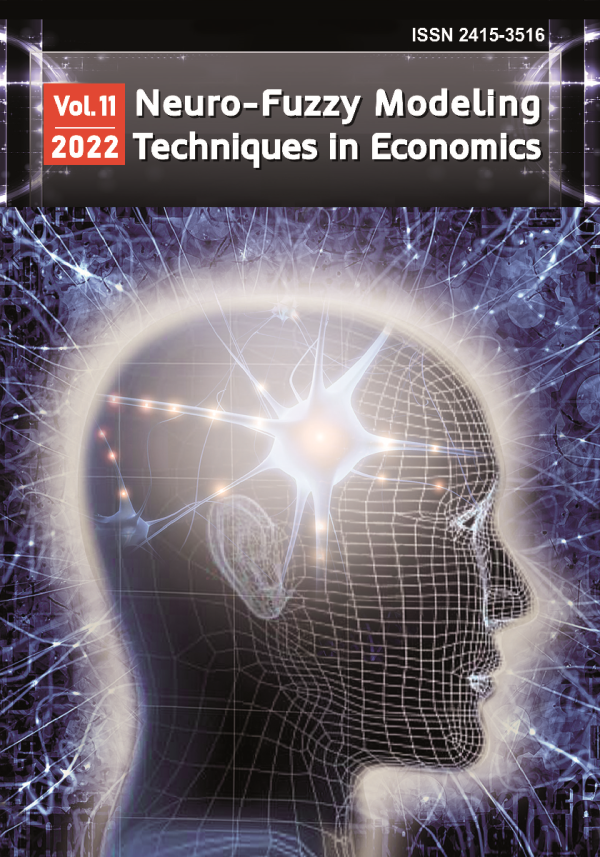
Neuro-Fuzzy Modeling Techniques in Economics
ISSN 2415-3516
Дослідження способів трансформації даних в контексті підвищення ефективності моделей кредитного скорингу
Studying the methods of data transformation in the context of increasing the effectiveness of credit scoring models
DOI:
10.33111/nfmte.2019.094
Анотація: У статті проведено дослідження з пошуку найефективнішого підходу до попередньої обробки характеристичних ознак позичальників з метою підвищення точності передбачення дефолтів за кредитними зобов’язаннями. Проаналізовано три основних способи подання даних на входи моделей кредитного скорингу: застосування початкових пояснюючих змінних без трансформації, переведення категоріальних характеристик у набір фіктивних змінних, біннінг показників із розрахунком вагомості ознаки (WOE) для кожної категорії.
Для отримання висновків щодо систематичного впливу цих підходів було проведено по 10 повторюваних ітерацій з побудови нейромережевих моделей персептронного типу за кожним із цих трьох способів підготовки вхідних факторів. Кожна скорингова модель оцінювалась за широким набором показників інтегральної та точкової ефективності.
Результати проведених експериментів засвідчили практично за всіма критеріями перевагу запропонованого автором методологічного підходу до попередньої обробки даних шляхом розбиття кількісних змінних на категорії із забезпеченням тренду їх показників вагомості ознаки та дотриманням обмежень на обсяг спостережень у кожній групі.
Abstract: The article highlights a study on the search for the most effective approach to pre-processing the characteristics of borrowers in order to improve the accuracy of predicting defaults on credit obligations. Three main ways of providing data to the inputs of credit scoring models are analyzed: the use of the initial explanatory variables without transformation, the conversion of categorical characteristics into a set of dummy variables, binning the indicators with the calculation of the weights of evidence (WOE) for each category.
To obtain conclusions about the systematic impact of these approaches, 10 repeated iterations were carried out with the construction of perceptron-type neural network models based on each of these three methods of preparing input factors. All scoring models were evaluated by a wide range of indicators of integrated and point efficiency.
The results of the experiments showed by almost all criteria the advantage of the methodological approach proposed by the author for preliminary data processing by dividing quantitative variables into categories, ensuring the trend in values of their weights of evidence and observing restrictions on the volume of observations in each group.
Ключові слова: скорингова модель, нейронна мережа, кредитоспроможність, біннінг, вагомість ознаки (WOE), інформаційна значущість (IV), коефіцієнт Джині.
Key words: scoring model, neural network, creditworthiness, binning, weight of evidence (WOE), informational value (IV), Gini coefficient.
УДК: 519.86+330.46
UDC: 519.86+330.46
JEL: C45 C51 C52 C53
To cite paper
In APA style
Kleban, Y. (2019). Studying the methods of data transformation in the context of increasing the effectiveness of credit scoring models. Neuro-Fuzzy Modeling Techniques in Economics, 8, 94-123. http://doi.org/10.33111/nfmte.2019.094
In MON style
Клебан Ю. Дослідження способів трансформації даних в контексті підвищення ефективності моделей кредитного скорингу. Нейро-нечіткі технології моделювання в економіці. 2019. № 8. С. 94-123. http://doi.org/10.33111/nfmte.2019.094 (дата звернення: 27.07.2024).
With transliteration
Kleban, Y. (2019) Doslidzhennia sposobiv transformatsii danykh v konteksti pidvyshchennia efektyvnosti modelei kredytnoho skorynhu [Studying the methods of data transformation in the context of increasing the effectiveness of credit scoring models]. Neuro-Fuzzy Modeling Techniques in Economics, no. 8. pp. 94-123. http://doi.org/10.33111/nfmte.2019.094 [in Ukrainian] (accessed 27 Jul 2024).
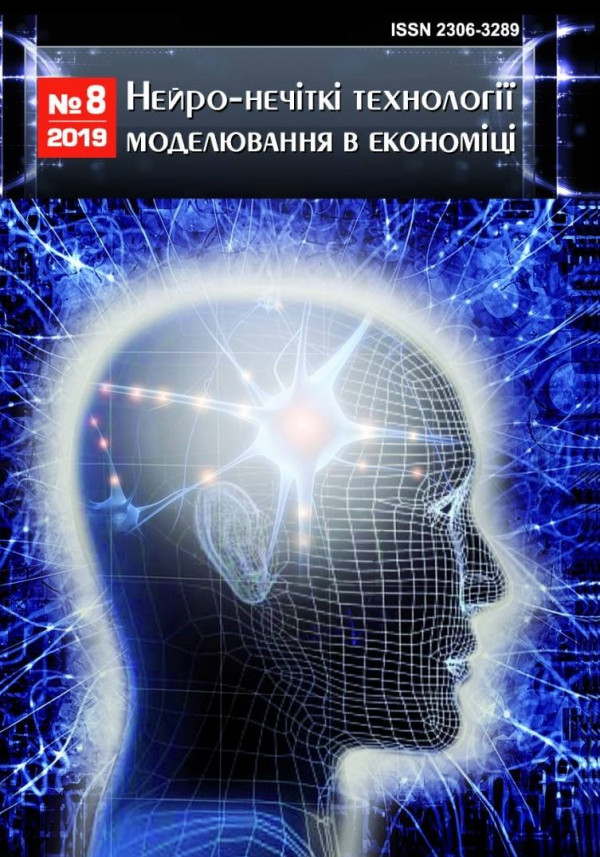
Download Paper
163
Views
42
Downloads
1
Cited by