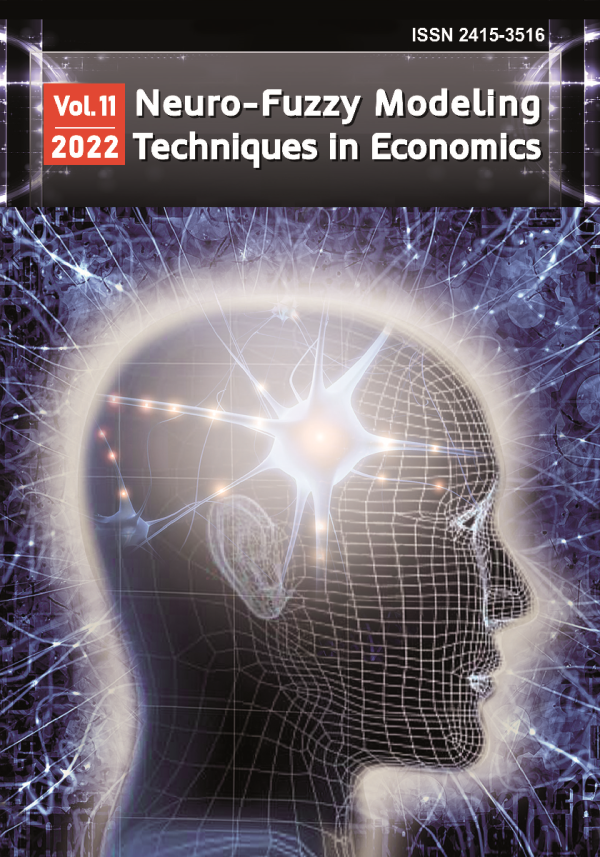
Neuro-Fuzzy Modeling Techniques in Economics
ISSN 2415-3516
Дослідження залежності ефективності моделювання кредитоспроможності позичальників від способу формування контрольної вибірки
Research of the dependence of the efficiency of modeling the creditworthiness of borrowers on the method of forming a control subset
DOI:
10.33111/nfmte.2020.156
Анотація: У статті проведено дослідження з підвищення стійкості результатів класифікації кредитоспроможності боржників комерційного банку з використанням бустингових дерев рішень та нейромережевих алгоритмів за рахунок застосування стратифікованого семплінгу. Запропоновано удосконалення класичної процедури стратифікованого семплінгу шляхом врахування при формуванні контрольної вибірки не тільки цільової змінної, але й найбільш значущих предикторів моделі.
Експериментальні розрахунки для перевірки висунутих гіпотез проведено з використанням програмних пакетів LGBM і H2O на даних міжнародного провайдера споживчого кредитування Home Credit. У статті перевірено та підтверджено, що використання стратифікованого семплінгу в процесі формування контрольної вибірки під час навчання моделей машинного навчання дозволяє підвищити їх стабільність і точність прогнозів на нових наборах даних.
Відповідно до отриманих результатів, авторський підхід до стратифікованого семплінгу при формуванні контрольного набору даних за цільовою змінною та найбільш значущими характеристиками моделі демонструє вищу середню точність для бустингових дерев рішень на тестовій вибірці в порівнянні зі стандартним стратифікованим алгоритмом семплінгу та випадковим відбором.
Abstract: In the article has been conducted a research aiming increase of classification result stability of commercial bank’s debtor creditworthiness with usage of boosted decision trees and neural network algorithms due to the use of stratified sampling. It is proposed to improve the classical procedure of stratified sampling by taking into account not only the target variable, but also the most significant predictors of the model when forming the control subset.
Experimental calculations to test the proposed hypotheses were carried out using the program packages LGBM and H2O on the data of international consumer finance provider Home Credit. In the article checked and confirmed that the use of stratified sampling in the process of forming a control subset during training of machine learning models makes possible to increase their stability and accuracy of forecasts on new data sets.
As per the achieved results, the authors’ approach of stratified sampling during forming a control dataset by target variable and the most significant characteristics of a model demonstrates a higher average accuracy for boosted decision trees on the test subset compared to the standard stratified sampling algorithm and random selection.
Ключові слова: дерево рішень, градієнтний бустинг, нейронна мережа, стратифікований семплінг
Key words: decision tree, gradient boosting, neural network, stratified sampling
УДК: 330.4
UDC: 330.4
JEL: C38 C45 C51 C52 C63
To cite paper
In APA style
Pyrohov, V., & Turchenko, S. (2020). Research of the dependence of the efficiency of modeling the creditworthiness of borrowers on the method of forming a control subset. Neuro-Fuzzy Modeling Techniques in Economics, 9, 156-174. http://doi.org/10.33111/nfmte.2020.156
In MON style
Пирогов В., Турченко С. Дослідження залежності ефективності моделювання кредитоспроможності позичальників від способу формування контрольної вибірки. Нейро-нечіткі технології моделювання в економіці. 2020. № 9. С. 156-174. http://doi.org/10.33111/nfmte.2020.156 (дата звернення: 21.05.2024).
With transliteration
Pyrohov, V., Turchenko, S. (2020) Doslidzhennia zalezhnosti efektyvnosti modeliuvannia kredytospromozhnosti pozychalnykiv vid sposobu formuvannia kontrolnoi vybirky [Research of the dependence of the efficiency of modeling the creditworthiness of borrowers on the method of forming a control subset]. Neuro-Fuzzy Modeling Techniques in Economics, no. 9. pp. 156-174. http://doi.org/10.33111/nfmte.2020.156 (accessed 21 May 2024).
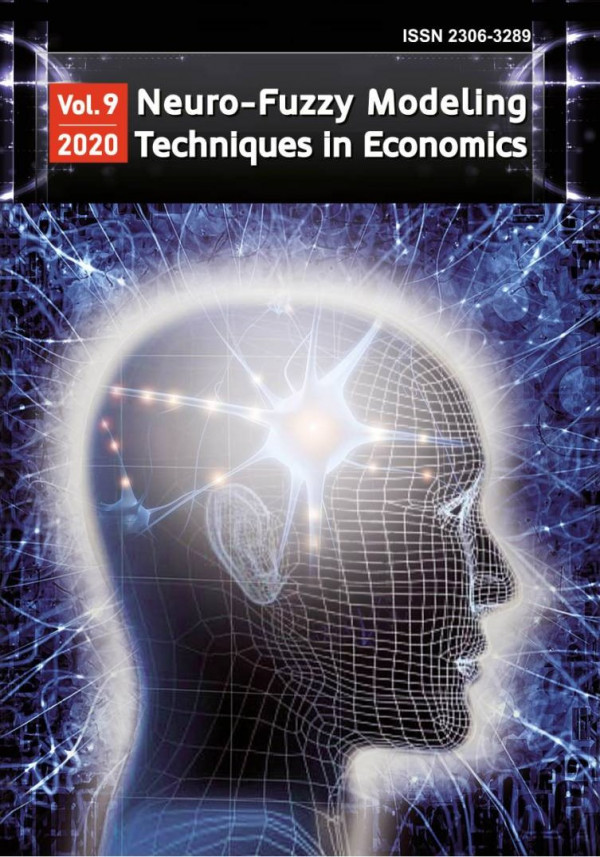
Download Paper
113
Views
17
Downloads
0
Cited by