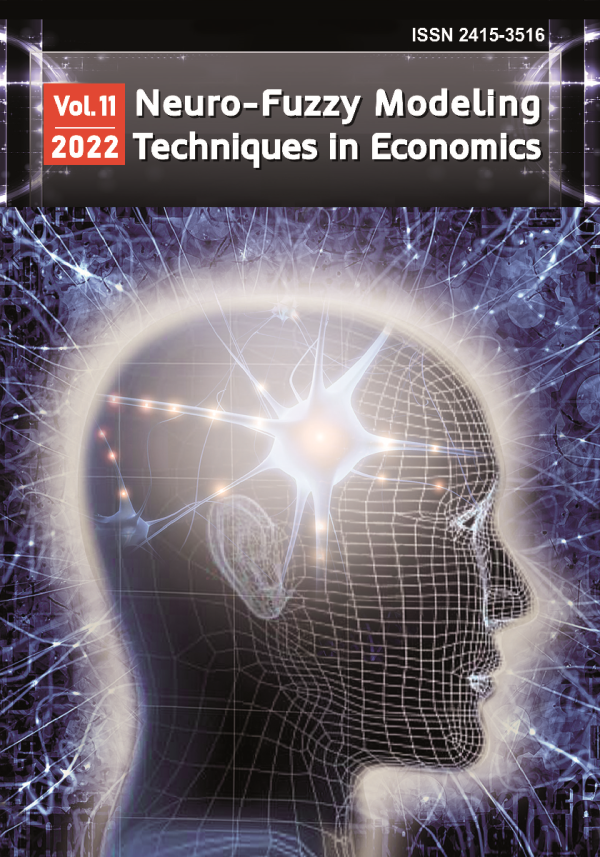
Neuro-Fuzzy Modeling Techniques in Economics
ISSN 2415-3516
Modeling national decarbonization capabilities using Kohonen maps
DOI:
10.33111/nfmte.2022.003
Анотація:
Abstract: This study sought to develop a method to cluster countries based on their decarbonization capabilities and to determine how these nations’ reduction of carbon dioxide (CO2) emissions has evolved over time. CO2 emissions clusters were identified using 11 indicators that measure both direct and indirect CO2 emissions, differentiating countries by their economic and population growth, energy consumption, and CO2 emission level. The panel data included 39 countries over the 10-year period of 2012–2021. The clustering was based on such type of neural networks as Kohonen self-organizing maps. This type of model facilitated grouping countries by similar decarbonization capabilities and economic development. The findings reveal that Norway and Sweden are the leaders in creating climate-resilient economies among the 39 countries analyzed. The analysis carried out can help other countries establish benchmarks for improving their own internal decarbonization activities based on leader nations’ strategies and borrowing their best practices for more efficient results.
This study thus contributes to the literature regarding decarbonization activities by offering a multi-country dynamic clustering method using Kohonen maps.
Ключові слова:
Key words: carbon dioxide (CO2), emission target, decarbonization, clustering, self-organizing map, neural network
УДК:
UDC:
JEL: C45 C53 Q53 Q56
To cite paper
In APA style
Zhytkevych, O., & Brochado, A. (2022). Modeling national decarbonization capabilities using Kohonen maps. Neuro-Fuzzy Modeling Techniques in Economics, 11, 3-24. http://doi.org/10.33111/nfmte.2022.003
In MON style
., Брочадо А. Modeling national decarbonization capabilities using Kohonen maps. Нейро-нечіткі технології моделювання в економіці. 2022. № 11. С. 3-24. http://doi.org/10.33111/nfmte.2022.003 (дата звернення: 27.07.2024).
With transliteration
Zhytkevych, O., Brochado, A. (2022) Modeling national decarbonization capabilities using Kohonen maps. Neuro-Fuzzy Modeling Techniques in Economics, no. 11. pp. 3-24. http://doi.org/10.33111/nfmte.2022.003 (accessed 27 Jul 2024).
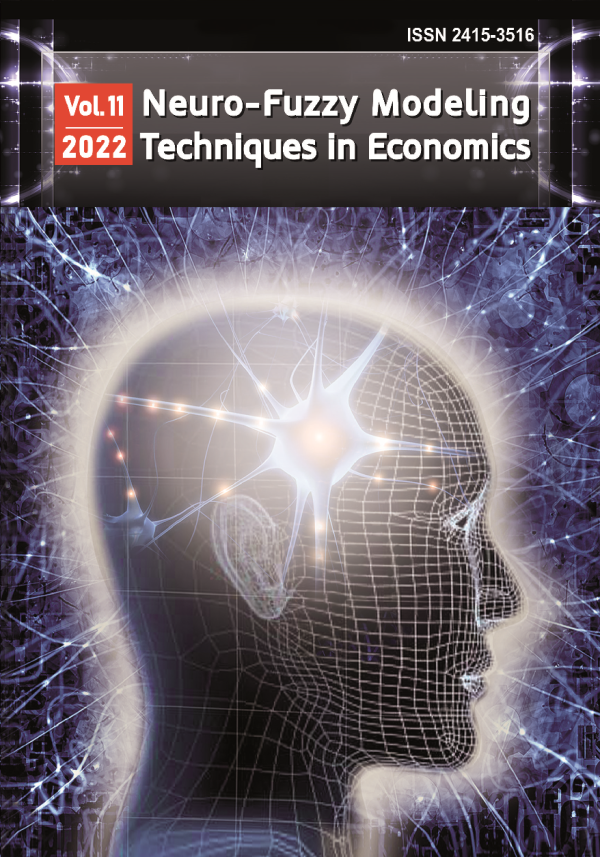
Download Paper
339
Views
104
Downloads
1
Cited by
- Enerdata. (2022). Global Energy & CO2 Data [Data set]. Retrieved July 1, 2022, from https://www.enerdata.net/research/energy-market-data-co2-emissions-database.html
- Publications Office of the European Union. (2019). A vision for the European industry until 2030: final report of the Industry 2030 high level industrial roundtable. European Commission. https://doi.org/10.2873/102179
- Churchill, S. A., Inekwe, J., & Ivanovski, K. (2018). Conditional convergence in per capita carbon emissions since 1900. Applied Energy, 228, 916-927. https://doi.org/10.1016/j.apenergy.2018.06.132
- Cai, Y., Sam, C.Y., & Chang, T. (2018). Nexus between clean energy consumption, economic growth and CO2 emissions. Journal of Cleaner Production, 182, 1001–1011. https://doi.org/10.1016/j.jclepro.2018.02.035
- Mrabet, Z., AlSamara, M., & Hezam Jarallah, S. (2017). The impact of economic development on environmental degradation in Qatar. Environmental and Ecological Statistics, 24(1), 7-38. https://doi.org/10.1007/s10651-016-0359-6
- Wu, T.-H., Chen, Y.-S., Shang, W., & Wu, J.-T. (2018). Measuring energy use and CO2 emission performances for APEC economies. Journal of Cleaner Production, 183, 590-601. https://doi.org/10.1016/j.jclepro.2018.02.028
- Zhou, W.-Y, Yang, W.-L., Wan, W.-X, Zhang, J., Zhou, W., Yang, H.-S., Yang, H., Xiao, H., Deng, S.-H., Shen, F., & Wang, Y.-J. (2018). The influences of industrial gross domestic product, urbanization rate, environmental investment, and coal consumption on industrial air pollutant emission in China. Environmental and Ecological Statistics, 25(4), 429-442. https://doi.org/10.1007/s10651-018-0412-8
- Li, D.-C., Chang, C.-J., Chen, C.-C., & Chen, W.-C. (2012). Forecasting short-term electricity consumption using the adaptive grey-based approach — an Asian case. Omega, 40(6), 767–773. https://doi.org/10.1016/j.omega.2011.07.007
- Schandl, H., Hatfield-Dodds, S., Wiedmann, T., Geschke, A., Cai, Y., West, J., Newth, D., Baynes, T., Lenzen, M., & Owen, A. (2016). Decoupling global environmental pressure and economic growth: scenarios for energy use, materials use and carbon emissions. Journal of Cleaner Production, 132, 45-56. https://doi.org/10.1016/j.jclepro.2015.06.100
- Al-mulali, U., Weng-Wai, C., Sheau-Ting, L., & Mohammed, A. H. (2015). Investigating the environmental Kuznets curve (EKC) hypothesis by utilizing the ecological footprint as an indicator of environmental degradation. Ecological Indicators, 48, 315–323. https://doi.org/10.1016/j.ecolind.2014.08.029
- He, Z., Xu, S, Shen, W., Long, R., & Chen, H. (2017). Impact of urbanization on energy related CO2 emission at different development levels: regional difference in China based on panel estimation. Journal of Cleaner Production, 140(3), 1719-1730. https://doi.org/10.1016/j.jclepro.2016.08.155
- Li, T., Wang, Y., & Zhao, D. (2016). Environmental Kuznets curve in China: New evidence from dynamic panel analysis. Energy Policy, 91, 138–147. https://doi.org/10.1016/j.enpol.2016.01.002
- Zi, C., Jie, W., & Hong-Bo, C. (2016). CO2 emissions and urbanization correlation in China based on threshold analysis. Ecological Indicators, 61(2), 193-201. https://doi.org/10.1016/j.ecolind.2015.09.013
- Appiah, K., Du, J., & Poku, J. (2018). Causal relationship between agricultural production and carbon dioxide emissions in selected emerging economies. Environmental Science and Pollution Research, 25, 24764-24777. https://doi.org/10.1007/s11356-018-2523-z
- Sarkodie, S. A., & Owusu, P. A. (2017). The relationship between carbon dioxide, crop and food production index in Ghana: By estimating the long-run elasticities and variance decomposition. Environmental Engineering Research, 22(2), 193-202. https://doi.org/10.4491/eer.2016.135
- Appiah, K., Du, J., Yeboah, M., & Appiah, R. (2019). Causal Correlation between Energy Use and Carbon Emissions in Selected Emerging Economies—Panel Model Approach. Environmental Science and Pollution Research, 26, 7896-7912. https://doi.org/10.1007/s11356-019-04140-2
- Apergis, N., & Payne, J. E. (2012). Renewable and Non-renewable Energy Consumption-Growth Nexus: Evidence from a Panel Error Correction Model. Energy Economics, 34(3), 733-738. https://doi.org/10.1016/j.eneco.2011.04.007
- Dogan, E., & Seker, F. (2016). Determinants of CO2 Emissions in the European Union: The Role of Renewable and Non-renewable Energy. Renewable Energy, 94, 429-439. https://doi.org/10.1016/j.renene.2016.03.078
- Asafu-Adjaye, J., Byrne, D., & Alvarez, M. (2016). Economic Growth, Fossil Fuel and Non-fossil Consumption: A Pooled Mean Group Analysis Using Proxies for Capital. Energy Economics, 60, 345-356. https://doi.org/10.1016/j.eneco.2016.10.016
- Boamah, K. B., Du, J., Boamah, A. J., & Appiah, K. (2018). A Study on the Causal Effect of Urban Population Growth and International Trade on Environmental Pollution: Evidence from China. Environmental Science and Pollution Research, 25, 5862-5874. https://doi.org/10.1007/s11356-017-0882-5
- Kim, D.-H., Suen, Y.-B., & Lin, S.-C. (2019). Carbon Dioxide Emissions and Trade: Evidence from Disaggregate Trade Data. Energy Economics, 78, 13-28. https://doi.org/10.1016/j.eneco.2018.08.019
- Xu, R, & Wunsch, D. (2005). Survey of Clustering Algorithms. IEEE Transaction on Neural Network, 16(3), 645-678. https://doi.org/10.1109/TNN.2005.845141
- Harkanth, S., & Phulpagar, B. D. (2013). A survey on clustering methods and algorithms. International Journal of Computer Science and Information Technologies, 4(5), 687-691. https://ijcsit.com/docs/Volume%204/Vol4Issue5/ijcsit2013040511.pdf
- Bini, B. S., & Mathew, T. (2016). Clustering and Regression Techniques for Stock Prediction. Procedia Technology, 24, 1248–1255. https://doi.org/10.1016/j.protcy.2016.05.104
- Lukianenko, D., & Strelchenko, I. (2021). Neuromodeling of features of crisis contagion on financial markets between countries with different levels of economic development. Neiro-Nechitki Tekhnolohii Modelyuvannya v Ekonomitsi (Neuro-Fuzzy Modeling Techniques in Economics), 10, 136-163. http://doi.org/10.33111/nfmte.2021.136
- Velykoivanenko, H., & Korchynskyi, V. (2022). Application of Clustering in the Dimensionality Reduction Algorithms for Separation of Financial Status of Commercial Banks in Ukraine. Universal Journal of Accounting and Finance, 10(1), 148-160. http://doi.org/10.13189/ujaf.2022.100116
- Gong, B., Zheng, X., Guo, Q., & Ordieres-Meré, J. (2019). Discovering the patterns of energy consumption, GDP, and CO2 emissions in China using the cluster method. Energy, 166, 1149-1167. https://doi.org/10.1016/j.energy.2018.10.143
- Csereklyei, Z., Anantharama, N., & Kallies, A. (2021). Electricity market transitions in Australia: Evidence using model-based clustering. Energy Economics, 103, Article 105590. https://doi.org/10.1016/j.eneco.2021.105590
- Inekwe, J., Maharaj, E. A. & Bhattacharya, M. (2020). Drivers of carbon dioxide emissions: an empirical investigation using hierarchical and non-hierarchical clustering methods. Environmental and Ecological Statistics, 27, 1–40. https://doi.org/10.1007/s10651-019-00433-4
- The World Bank. (2022). World Development Indicators [Data set]. Retrieved July 1, 2022, from https://datacatalog.worldbank.org/search/dataset/0037712
- United Nations Statistics Division. (2022). UNdata [Data set]. Retrieved July 1, 2022, from http://data.un.org/Explorer.aspx
- United Nations Climate Change. (2022). Time Series - Annex I [Data set]. Retrieved July 1, 2022, from https://di.unfccc.int/time_series
- International Renewable Energy Agency. (2022). Statistics Data [Data set]. Retrieved July 1, 2022, from https://irena.org/Statistics
- Eurostat. (2022). DATABASE [Data set]. Retrieved July 1, 2022, from https://ec.europa.eu/eurostat/en/web/main/data/database
- Enerdata. (2022). World Energy & Climate Statistics – Yearbook 2022 [Data set]. Retrieved July 1, 2022, from https://yearbook.enerdata.net/total-energy/world-consumption-statistics.html
- Kohonen, T. (2001). Springer Series in Information Sciences: Vol. 30. Self-organizing maps (3rd ed.). Springer. https://doi.org/10.1007/978-3-642-56927-2
- Wehrens, R., & Kruisselbrink, J. (2018). Flexible Self-Organizing Maps in kohonen 3.0. Journal of Statistical Software, 87(7), 1-18. https://doi.org/10.18637/jss.v087.i07
- Matviychuk, A., Lukianenko, O., & Miroshnychenko, I. (2019). Neuro-fuzzy model of country’s investment potential assessment. Fuzzy economic review, 24(2), 65-88. https://doi.org/10.25102/fer.2019.02.04
- Food and Agriculture Organization of the United Nations. (2020). Intended Nationally Determined Contribution (INDC) of Ukraine to a New Global Climate Agreement. https://www.fao.org/faolex/results/details/en/c/LEX-FAOC190395/
- UNICEF. (2021). Climate Landscape Analysis for Children (CLAC) in Ukraine. https://www.unicef.org/ukraine/media/15766/file/Climate%20Landscape%20Analysis%20for%20Children%20(CLAC)%20in%20Ukraine.pdf
- Ministry of Ecology and Natural Resources of Ukraine. (2021). Analytical review of the updated nationally determined contribution of Ukraine to the Paris agreement. https://mepr.gov.ua/files/docs/klimatychna_polityka/Analytical%20Review%20Updated%20Nationally%20Determined%20Contribution%20of%20Ukraine%20to%20the%20Paris%20Agreement%20July%202021%20(2).pdf