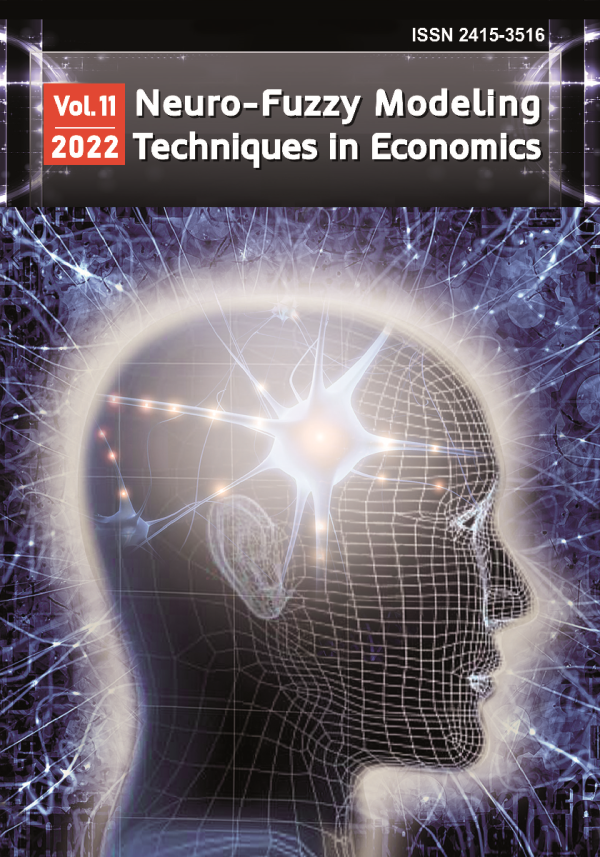
Neuro-Fuzzy Modeling Techniques in Economics
ISSN 2415-3516
Modeling the values of reflexive characteristics of agents within the management of herd behavior at the enterprises
DOI:
10.33111/nfmte.2022.048
Анотація:
Abstract: The central place at the formation of agent behavior at enterprises is the study of decision-making procedures and factors that mediate their choice. To determine the values of the reflexive characteristics of agents in the framework of management of herd behavior at enterprises a new approach is proposed based on questionnaire methods, the apparatus of the theory of fuzzy sets and neural network modeling.
The determination of the values of reflexive characteristics of agents is carried out by the formation of fuzzy sets in the framework of the theory of L. Zadeh based on the results of a questionnaire of agents in selected areas. The agents are distributed by the Kohonen map into groups in order to numerically determine the values of their reflexive characteristics based on formed fuzzy sets. An important applied value in interpreting the results of the Kohonen SOM clustering is the ability to obtain representatives of specific clusters and average values of their characteristics, which are determined by the parameters of the network neurons and represent cluster centers. As a result of the clustering of input data vectors by directions of determining the values of the reflexive characteristics of agents, typical values of the required parameters are obtained for agents-representatives of clusters. The values of the reflexive characteristics of agents can be used to evaluate the results of decision-making by agents using the functions of reflexive choice to ensure effective management of manifestations of herd behavior at enterprises.
The proposed modeling methodology will allow to identify the prerequisites for the manifestation of herd behavior at enterprises and the potential circle of agents for the formation of adequate managing actions in the process of ensuring effective management of herd behavior and achieving the goals of the enterprise.
Ключові слова:
Key words: modeling, reflexive characteristics, agent, herd behavior, enterprise, questionnaires, fuzzy sets, self-organizing map
УДК:
UDC:
JEL: C02 C45 C52 C53 D91
To cite paper
In APA style
Turlakova, S. (2022). Modeling the values of reflexive characteristics of agents within the management of herd behavior at the enterprises. Neuro-Fuzzy Modeling Techniques in Economics, 11, 48-77. http://doi.org/10.33111/nfmte.2022.048
In MON style
Турлакова С. Modeling the values of reflexive characteristics of agents within the management of herd behavior at the enterprises. Нейро-нечіткі технології моделювання в економіці. 2022. № 11. С. 48-77. http://doi.org/10.33111/nfmte.2022.048 (дата звернення: 27.07.2024).
With transliteration
Turlakova, S. (2022) Modeling the values of reflexive characteristics of agents within the management of herd behavior at the enterprises. Neuro-Fuzzy Modeling Techniques in Economics, no. 11. pp. 48-77. http://doi.org/10.33111/nfmte.2022.048 (accessed 27 Jul 2024).
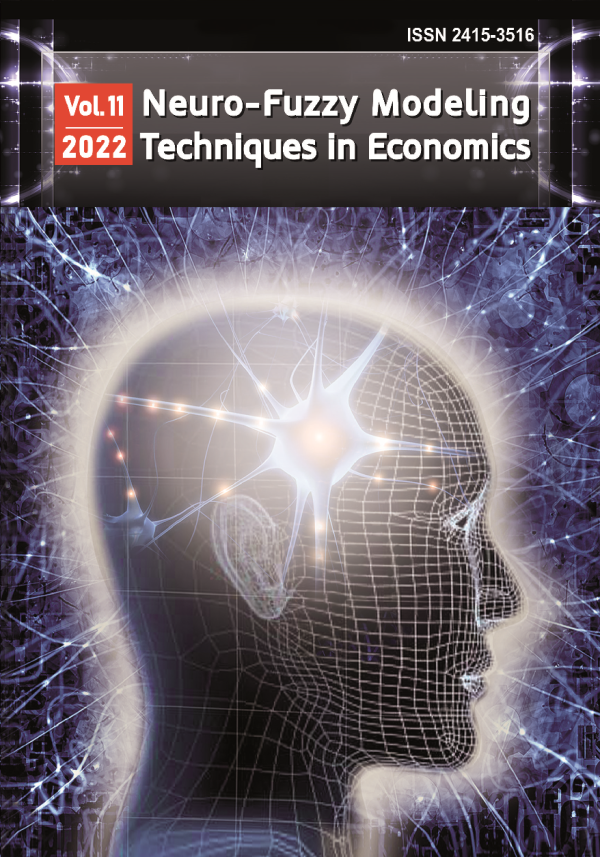
Download Paper
210
Views
61
Downloads
1
Cited by
- Allais, M. (1953). Le comportement de l’homme rationnel devant le risque: critique des postulats et axiomes de l’école américaine. Econometrica, 21(2), 503–549.
- Kahneman, D., & Tversky, А. (1979). Prospect theory: an analysis of decisions under risk. Econometrica, 47(2), 263-291. https://doi.org/10.2307/1914185
- Thaler, R. H. (2015). Misbehaving: The Making of Behavioral Economics. W.W. Norton & Company.
- Yi., H., & Xiugang, Y. (2019). Managers’ Overconfidence, Risk Preference, Herd Behavior and Non-efficient Investment. Independent Journal of Management & Production, 10(1), 56-75. https://doi.org/10.14807/ijmp.v10i1.845
- Loxton, M., Truskett, R., Scarf, B., Sindone, L., Baldry, G., & Zhao, Y. (2020). Consumer behaviour during crises: Preliminary research on how coronavirus has manifested consumer panic buying, herd mentality, changing discretionary spending and the role of the media in influencing behaviour. Journal of risk and financial management, 13(8), Article 166. https://doi.org/10.3390/jrfm13080166
- Pavlović-Höck, N. (2022). Herd behaviour along the consumer buying decision process - experimental study in the mobile communications industry. Digital Business, 2(1), Article 100018. https://doi.org/10.1016/j.digbus.2021.100018
- Fang, H., Lu, Y.-Ch., Shieh, J.C.P., & Lee, Y.-Hs. (2021). The existence and motivations of irrational loan herding and its impact on bank performance when considering different market periods. International Review of Economics & Finance, 73, 420-443. https://doi.org/10.1016/j.iref.2021.01.015
- Merkulova, T., & Kononova, K. (2015). Neyrosetevoy podhod k modelirovaniyu povedeniya: analiz rezultatov eksperimenta «Obschestvennoe blago» [Neural network approach to modeling behavior: the analysis of PG game outcomes]. Neiro-nechitki tekhnolohii modeliuvannia v ekonomitsi (Neuro-Fuzzy Modeling Techniques in Economics), 4, 113–134. http://doi.org/10.33111/nfmte.2015.113 [in Russian]
- Danich, V.M., & Shekhovtsova, K.V. (2013). Valiutna panika, azhiotazh ta yikh vplyv na diialnist pidpryiemstv [Currency Panic, Agiotage and their Influence on Company Activity]. Biznes Inform (Business Inform), 6, 8–13. https://www.business-inform.net/export_pdf/business-inform-2013-6_0-pages-8_13.pdf [in Ukrainian]
- Solodukhin, S. V. (2017). Osnovnyie predposylky postroeniya modeley stadnoho povedeniya vo vneshney i vnutrenney srede predpriyatiy [The main prerequisites for the construction of models of herd behavior in the external and internal environment of enterprises]. Problemy systemnoho pidkhodu v ekonomitsi (Problems of the systemic approach in economy), 4(60), 181–186. http://www.psae-jrnl.nau.in.ua/journal/4_60_2017_ukr/26.pdf [in Russian]
- Lepa, R. M., Okhten, A.A., & Stashkevich, I.I. (2016). Minimizatsiia oporu personalu orhanizatsiinym zminam na pidpryiemstvi [Minimizing personnel resistance to organizational changes at enterprise]. Ekonomika promyslovosti (Economy of industry), 3(75), 90–115. https://doi.org/10.15407/econindustry2016.03.090 [in Ukrainian]
- Stashkevych, I., Turlakova, S., Shevchenko, O., & Derzhevetska, M. (2020). IDEF0-Technology of Modeling of Processes of Minimization the Resistance of the Personnel to Organizational Changes at the Enterprise. WSEAS Transactions on Environment and Development, 16, 286-296. https://doi.org/10.37394/232015.2020.16.30
- Turlakova, S., Vyshnevskyi, O., Lohvinenko, B., & Fomichenko, I. (2020). Role of Reflexive Characteristics of Agents in the Level of Consistency of Goals in the Group. WSEAS Transactions on Environment and Development, 16, 297–304. https://doi.org/10.37394/232015.2020.16.31
- Turlakova, S.S. (2020). Refleksivnoe upravlenie stadnyim povedeniem na predpriyatiyah: kontseptsiya, modeli i metodyi (Reflexive management of herd behavior at enterprises: concept, models and methods). NAN Ukrainy, In-t ekonomiki prom-sti. [in Russian]
- Zadeh, L.A. (2008). Is there a need for fuzzy logic? Information Sciences, 178(13), 2751-2779. https://doi.org/10.1016/j.ins.2008.02.012
- Cattell, R. B. (1988). The meaning and strategic use of factor analysis. In J. R. Nesselroade & R. B. Cattell (Eds.), Handbook of Multivariate Experimental Psychology (pp. 131–203). Plenum Press. https://doi.org/10.1007/978-1-4613-0893-5_4
- McClelland, D. C. (2001). Chapter 27: Where Do We Stand on Assessing Competencies? In J. Raven, & J. Stephenson (Eds.), Counterpoints: Vol. 166. Competence in the learning society (pp. 479–489). Peter Lang AG. https://www.jstor.org/stable/42977810
- Spencer, L. M., & Spencer, S. M. (2008). Competence at Work models for superior performance. John Wiley & Sons.
- Hartigan, J.A., & Wong, M.A. (1979). A k-means clustering algorithm. Journal of the Royal Statistical Society. Series C, 28(1), 100-108. https://doi.org/10.2307/2346830
- Kaufman, L., & Rousseeuw, P. J. (1990). Partitioning Around the Medoids (Program PAM). In Wiley Series in Probability and Statistics. Finding Groups in Data: An Introduction to Cluster Analysis (pp. 68–125). John Wiley & Sons. https://doi.org/10.1002/9780470316801.ch2
- Jolliffe, I.T. (2002). Principal Component Analysis (2nd ed.). Springer. https://link.springer.com/book/10.1007/b98835
- van der Maaten, L., & Hinton, G. E. (2008). Visualizing High-Dimensional Data Using t-SNE, Journal of Machine Learning Research, 9, 2579-2605. https://lvdmaaten.github.io/publications/papers/JMLR_2008.pdf
- Sokal, R., & Rohlf, F. (1962). The comparison of dendrograms by objective methods. Taxon, 11, 33-40. https://doi.org/10.2307/1217208
- Caliński, T., & Harabasz, J. (1974). A Dendrite Method for Cluster Analysis. Communications in Statistics - Theory and Methods, 3, 1-27. https://doi.org/10.1080/03610927408827101
- Schubert, E., Sander, J., Ester, M., Kriegel, H., & Xu, X. (2017). DBSCAN revisited, revisited: Why and how you should (still) use DBSCAN. ACM Transactions on Database Systems, 42(3), 1-21. https://doi.org/10.1145/3068335
- McInnes, L., & Healy, J. (2018). UMAP: Uniform Manifold Approximation and Projection for Dimension Reduction. arXiv. https://doi.org/10.48550/arXiv.1802.03426
- Zhang, T., Ramakrishnan, R., & Livny, M. (1996). BIRCH: an efficient data clustering method for very large databases. In Proceedings of the 1996 ACM SIGMOD international conference on Management of data (pp. 103–114). ACM. https://doi.org/10.1145/233269.233324
- Kohonen, T. (2001). Self-Organizing Maps. Springer–Verlag.
- Kohonen, T. (2008). Data management by self-organizing maps. In J.M. Zurada, G.G. Yen, & J. Wang (Eds.), Lecture Notes in Computer Science: Vol. 5050. Congress on Computational Intelligence: Research Frontiers (pp. 309-332). Springer. https://doi.org/10.1007/978-3-540-68860-0_15
- Kohonen, T. (2013). Essentials of the self-organizing map. Neural Networks, 37, 52-65. https://doi.org/10.1016/j.neunet.2012.09.018
- Mariño, L., & de Carvalho, F. (2022). Two weighted c-medoids batch SOM algorithms for dissimilarity data. Information Sciences, 607, 603-619. https://doi.org/10.1016/j.ins.2022.06.019
- Kobets, V., & Novak, O. (2021). EU countries clustering for the state of food security using machine learning techniques. Neuro-Fuzzy Modeling Techniques in Economics, 10, 86-118. https://doi.org/10.33111/nfmte.2021.086
- Kobets, V., & Yatsenko, V. (2019). Influence of the fourth industrial revolution on divergence and convergence of economic inequality for various countries. Neiro-Nechitki Tekhnolohii Modelyuvannya v Ekonomitsi (Neuro-Fuzzy Modeling Techniques in Economics), 8, 124-146. http://doi.org/10.33111/nfmte.2019.124
- Subasi, A. (2020). Clustering examples. In Practical Machine Learning for Data Analysis Using Python (pp. 465-511). Elsevier. http://doi.org/10.1016/b978-0-12-821379-7.00007-2
- Kaski, S. (2011). Self-Organizing Maps. In C. Sammut, G.I. Webb (Eds.), Encyclopedia of Machine Learning (pp. 886-888). Springer. https://doi.org/10.1007/978-0-387-30164-8_746
- Matviychuk, A., Lukianenko, O., & Miroshnychenko, I. (2019). Neuro-fuzzy model of country’s investment potential assessment. Fuzzy economic review, 24(2), 65-88. https://doi.org/10.25102/fer.2019.02.04
- Lo, Z.-P., Fujita, M., & Bavarian, B. (1991). Analysis of neighborhood interaction in Kohonen neural networks. In 6th International Parallel Processing Symposium Proceedings (pp. 247–249). IEEE Computer Society.