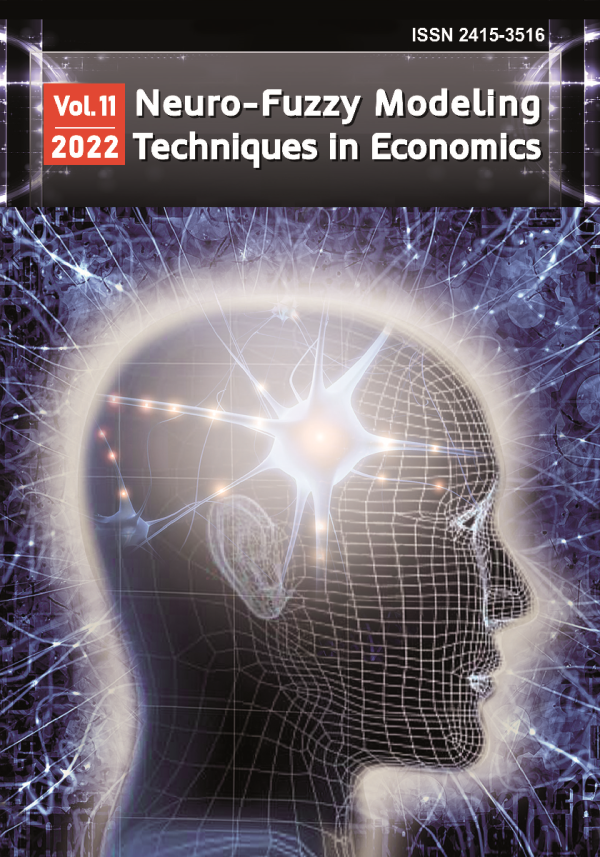
Neuro-Fuzzy Modeling Techniques in Economics
ISSN 2415-3516
Fuzzy time series forecasting using semantic artificial intelligence tools
DOI:
10.33111/nfmte.2022.157
Анотація:
Abstract: This study investigates the application of Fuzzy Time Series (FTS) methods in forecasting the Bitcoin market. FTS methods have gained significant attention due to their simplicity, adaptability, forecasting precision, and computational efficiency. They generate interpretable representations of time series patterns, enabling knowledge transfer, auditability, reusability, and upgradability. The study specifically focuses on time-invariant rule-based FTS techniques, namely the conventional First-Order FTS (Song and Chen) and Weighted First-Order FTS (Yu) models. The research rigorously evaluates and compares the predictive performance of these methods across a range of accuracy metrics. Additionally, the article expands the understanding and application of FTS methods in cryptocurrency forecasting. Through comprehensive experimental evaluations and statistical analyses, it uncovers insights into the strengths, limitations, and potential areas for improvement of these FTS approaches. By highlighting their comparative accuracy and computational efficiency, the research contributes to the existing studies and provides practical recommendations for researchers and practitioners in the cryptocurrency domain.
Ключові слова:
Key words: fuzzy time series, fuzzy set, fuzzy logical relationships group, fuzzy time series forecasting, Bitcoin, cryptocurrency market
УДК:
UDC:
JEL: C53 C63 G10
To cite paper
In APA style
Bielinskyi, A., Soloviev, V., Solovieva, V., & Velykoivanenko, H. (2022). Fuzzy time series forecasting using semantic artificial intelligence tools. Neuro-Fuzzy Modeling Techniques in Economics, 11, 157-198. http://doi.org/10.33111/nfmte.2022.157
In MON style
Белінський А., Соловйов В.М., Соловйова В., Великоіваненко Г.І. Fuzzy time series forecasting using semantic artificial intelligence tools. Нейро-нечіткі технології моделювання в економіці. 2022. № 11. С. 157-198. http://doi.org/10.33111/nfmte.2022.157 (дата звернення: 26.04.2025).
With transliteration
Bielinskyi, A., Soloviev, V., Solovieva, V., Velykoivanenko, H. (2022) Fuzzy time series forecasting using semantic artificial intelligence tools. Neuro-Fuzzy Modeling Techniques in Economics, no. 11. pp. 157-198. http://doi.org/10.33111/nfmte.2022.157 (accessed 26 Apr 2025).
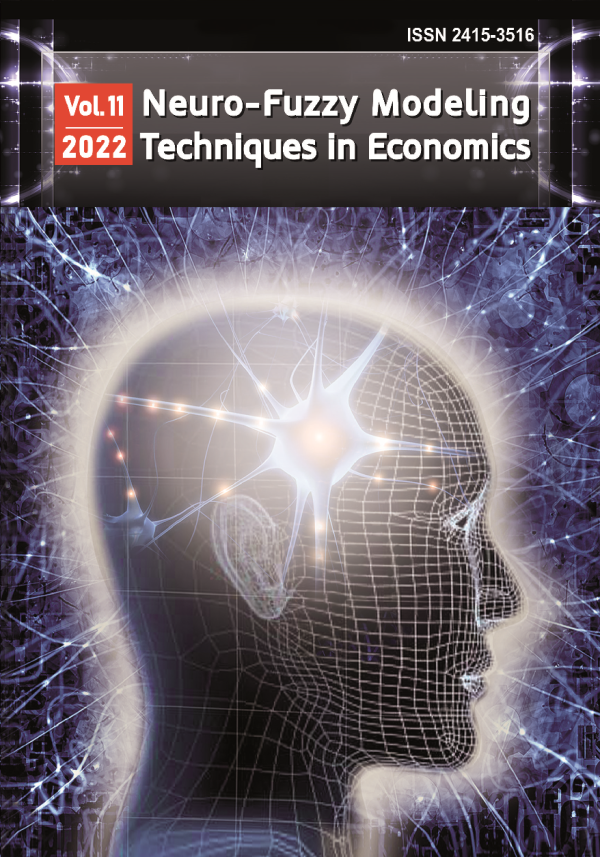
Download Paper
628
Views
166
Downloads
1
Cited by
- Bajestani, N. S., & Zare, A. (2011). Forecasting TAIEX using improved type 2 fuzzy time series. Expert Systems with Applications, 38(5), 5816–5821. https://doi.org/10.1016/j.eswa.2010.10.049
- Bielinskyi, A., & Soloviev, V. (2018). Complex network precursors of crashes and critical events in the cryptocurrency market. CEUR Workshop Proceeding, 2292, 37-45. https://ceur-ws.org/Vol-2292/paper02.pdf
- Bielinskyi, A., Hushko, S., Matviychuk, A., Serdyuk, O., Semerikov, S., & Soloviev, V. (2021). Irreversibility of financial time series: a case of crisis. CEUR Workshop Proceedings, 3048, 134-150. https://ceur-ws.org/Vol-3048/paper04.pdf
- Bielinskyi, A., Serdyuk, O., Semerikov, S., & Soloviev, V. (2021). Econophysics of cryptocurrency crashes: a systematic review. CEUR Workshop Proceedings, 3048, 31-133. https://ceur-ws.org/Vol-3048/paper03.pdf
- Bielinskyi, A., Soloviev, V., Semerikov, S., & Solovieva, V. (2021). Identifying stock market crashes by fuzzy measures of complexity. Neuro-Fuzzy Modeling Techniques in Economics, 10, 3-45. http://doi.org/10.33111/nfmte.2021.003
- Bielinskyi, A.O., Matviychuk, A.V., Serdyuk, O.A., Semerikov, S.O., Solovieva, V.V., & Soloviev, V.N. (2022). Correlational and Non-extensive Nature of Carbon Dioxide Pricing Market. In O. Ignatenko, V. Kharchenko, V. Kobets, et al. (Eds.). Communications in Computer and Information Science: Vol. 1635. ICTERI 2021 Workshops (pp. 183–199). Springer, Cham. https://doi.org/10.1007/978-3-031-14841-5_12
- Bitencourt, H. V., & Guimarães, F. G. (2021). High-dimensional Multivariate Time Series Forecasting in IoT Applications using Embedding Non-stationary Fuzzy Time Series. In Proceedings of 2021 IEEE Latin American Conference on Computational Intelligence (pp. 1-6). IEEE. https://doi.org/10.1109/la-cci48322.2021.9769792
- Bose, M., & Mali, K. (2018). A novel data partitioning and rule selection technique for modeling high-order fuzzy time series. Applied Soft Computing, 63, 87–96. https://doi.org/10.1016/j.asoc.2017.11.011
- Box, G. E. P., & Pierce, D. A. (1970). Distribution of Residual Autocorrelations in Autoregressive-Integrated Moving Average Time Series Models. Journal of the American Statistical Association, 65(332), 1509-1526. https://doi.org/10.2307/2284333
- Chen, M. Y. (2014). A high-order fuzzy time series forecasting model for internet stock trading. Future Generation Computer Systems, 37, 461–467. https://doi.org/10.1016/j.future.2013.09.025
- Chen, S. (1996). Forecasting enrollments based on fuzzy time series. Fuzzy Sets and Systems, 81(3), 311–319. https://doi.org/10.1016/0165-0114(95)00220-0
- Chen, S., & Chang, Y. (2010). Multi-variable fuzzy forecasting based on fuzzy clustering and fuzzy rule interpolation techniques. Information Sciences, 180(24), 4772–4783. https://doi.org/10.1016/j.ins.2010.08.026
- De Lima E Silva, P. C., Severiano, C. A., Alves, M. A., Silva, R., Cohen, M. W., & Guimarães, F. G. (2020). Forecasting in non-stationary environments with fuzzy time series. Applied Soft Computing, 97(B), Article 106825. https://doi.org/10.1016/j.asoc.2020.106825
- De Lima E Silva, P.C. (2019). Scalable Models For Probabilistic Forecasting With Fuzzy Time Series [Doctoral dissertation, Universidade Federal de Minas Gerais]. Zenodo. https://doi.org/10.5281/zenodo.3449195
- De Oliveira E Lucas, P., Orang, O., De Lima E Silva, P. C., Mendes, E., & Guimarães, F. G. (2021). A Tutorial on Fuzzy Time Series Forecasting Models: Recent Advances and Challenges. Learning and Nonlinear Models, 19(2), 29–50. https://doi.org/10.21528/lnlm-vol19-no2-art3
- Derbentsev, V., Matviychuk, A., & Soloviev, V.N. (2020). Forecasting of Cryptocurrency Prices Using Machine Learning. In L. Pichl, C. Eom, E. Scalas, & T. Kaizoji (Eds.), Advanced Studies of Financial Technologies and Cryptocurrency Markets (pp. 211-231). Springer, Singapore. https://doi.org/10.1007/978-981-15-4498-9_12
- Derbentsev, V., Velykoivanenko, H., & Datsenko, N. (2019). Machine learning approach for forecasting cryptocurrencies time series. Neuro-Fuzzy Modeling Techniques in Economics, 8, 65-93. http://doi.org/10.33111/nfmte.2019.065
- Derbentsev, V., Bezkorovainyi, V., Silchenko, M., Hrabariev, A., & Pomazun, O. (2021). Deep Learning Approach for Short-Term Forecasting Trend Movement of Stock Indeces. In 2021 IEEE 8th International Conference on Problems of Infocommunications, Science and Technology (PIC S&T) (pp. 607-612). IEEE. https://doi.org/10.1109/PICST54195.2021.9772235
- Dickey, D. A., & Fuller, W. A. (1979). Distribution of the Estimators for Autoregressive Time Series With a Unit Root. Journal of the American Statistical Association, 74(366), 427–431. https://doi.org/10.2307/2286348
- Dong, Q., & Ma, X. (2021). Enhanced fuzzy time series forecasting model based on hesitant differential fuzzy sets and error learning. Expert Systems with Applications, 166, Article 114056. https://doi.org/10.1016/j.eswa.2020.114056
- Dos Santos, M. M., Guimarães, F. G., & De Lima E Silva, P. C. (2021). High-dimensional Multivariate Time Series Forecasting using Self-Organizing Maps and Fuzzy Time Series. In Proceedings of 2021 IEEE International Conference on Fuzzy Systems (FUZZ-IEEE) (pp. 1-6). IEEE. https://doi.org/10.1109/fuzz45933.2021.9494496
- Dubois, D., & Prade, H. (1991). Fuzzy sets in approximate reasoning, Part 1: Inference with possibility distributions. Fuzzy Sets and Systems, 40(1), 143–202. https://doi.org/10.1016/0165-0114(91)90050-z
- Egrioglu, E., Aladag, C. H., Yolcu, U., Basaran, M. A., & Uslu, V. R. (2009). A new hybrid approach based on SARIMA and partial high order bivariate fuzzy time series forecasting model. Expert Systems with Applications, 36(4), 7424–7434. https://doi.org/10.1016/j.eswa.2008.09.040
- Egrioglu, E., Aladag, C. H., Yolcu, U., Uslu, V. R., & Basaran, M. A. (2009). A new approach based on artificial neural networks for high order multivariate fuzzy time series. Expert Systems with Applications, 36(7), 10589–10594. https://doi.org/10.1016/j.eswa.2009.02.057
- Egrioglu, E., Bas, E., Yolcu, U., & Chen, M. Y. (2020). Picture fuzzy time series: Defining, modeling and creating a new forecasting method. Engineering Applications of Artificial Intelligence, 88, Article 103367. https://doi.org/10.1016/j.engappai.2019.103367
- Huarng, K. (2001). Heuristic models of fuzzy time series for forecasting. Fuzzy Sets and Systems, 123(3), 369–386. https://doi.org/10.1016/s0165-0114(00)00093-2
- Hwang, J.-R., Chen, S.-M., & Lee, C.-H. (1998). Handling forecasting problems using fuzzy time series. Fuzzy Sets and Systems, 100(1–3), 217–228. https://doi.org/10.1016/s0165-0114(97)00121-8
- Ivanchenko, H., & Vashchaiev, S. (2018). Studying the dynamics of nonlinear interaction between enterprise populations. Neuro-Fuzzy Modeling Techniques in Economics, 7, 78-110. http://doi.org/10.33111/nfmte.2018.078
- Jin, X., Wang, Y.-X., & Yan, X. (2021). Inter-Series Attention Model for COVID-19 Forecasting. In C. Demeniconi, I. Davidson, L. Akoglu, & E. Terzi (Eds.), Proceedings of the 2021 SIAM International Conference on Data Mining (pp. 495–503). SIAM. https://doi.org/10.1137/1.9781611976700.56
- Kaminskyi, A., Miroshnychenko, I., & Pysanets, K. (2019). Risk and return for cryptocurrencies as alternative investment: Kohonen maps clustering. Neuro-Fuzzy Modeling Techniques in Economics, 8, 175-193. http://doi.org/10.33111/nfmte.2019.175
- Kmytiuk, T., & Majore, G. (2021). Time series forecasting of agricultural product prices using Elman and Jordan recurrent neural networks. Neuro-Fuzzy Modeling Techniques in Economics, 10, 67-85. http://doi.org/10.33111/nfmte.2021.067
- Kobets, V., & Novak, O. (2021). EU countries clustering for the state of food security using machine learning techniques. Neuro-Fuzzy Modeling Techniques in Economics, 10, 86-118. http://doi.org/10.33111/nfmte.2021.086
- Kozlovskyi, S., Mazur, H., Vdovenko, N., Shepel, T., & Kozlovskyi, V. (2018). Modeling and forecasting the level of state stimulation of agricultural production in Ukraine based on the theory of fuzzy logic. Montenegrin Journal of Economics, 14(3), 37-53. https://doi.org/10.14254/1800-5845/2018.14-3.3
- Lara-Benítez, P., Carranza-García, M., & Riquelme, J. C. (2021). An Experimental Review on Deep Learning Architectures for Time Series Forecasting. International Journal of Neural Systems, 31(03), Article 2130001. https://doi.org/10.1142/s0129065721300011
- Lee, C.-H. L., Liu, A., & Chen, W.-S. (2006). Pattern discovery of fuzzy time series for financial prediction. IEEE Transactions on Knowledge and Data Engineering, 18(5), 613–625. https://doi.org/10.1109/tkde.2006.80
- Liu, Y., Wu, H., Wang, J., & Long, M. (2022). Non-stationary transformers: Rethinking the stationarity in time series forecasting. arXiv. https://arxiv.org/abs/2205.14415
- Ljung, G. M., & Box, G. E. P. (1978). On a measure of lack of fit in time series models. Biometrika, 65(2), 297–303. https://doi.org/10.1093/biomet/65.2.297
- Luo, C. B., & Wang, H. (2020). Fuzzy forecasting for long-term time series based on time-variant fuzzy information granules. Applied Soft Computing, 88, Article 106046. https://doi.org/10.1016/j.asoc.2019.106046
- Matviychuk, A. (2006). Fuzzy logic approach to identification and forecasting of financial time series using Elliott wave theory. Fuzzy economic review, 11(2), 51-68. https://doi.org/10.25102/fer.2006.02.04
- Matviychuk, A., Lukianenko, O., & Miroshnychenko, I. (2019). Neuro-fuzzy model of country’s investment potential assessment. Fuzzy economic review, 24(2), 65-88. https://doi.org/10.25102/fer.2019.02.04
- Matviychuk, A., Strelchenko, I., Vashchaiev, S., & Velykoivanenko, H. (2019). Simulation of the Crisis Contagion Process Between Countries with Different Levels of Socio-Economic Development. CEUR Workshop Proceedings, 2393, 485-496. http://ceur-ws.org/Vol-2393/paper_423.pdf
- Olivares, K. G., Challu, C., Marcjasz, G., Weron, R., & Dubrawski, A. (2021). Neural basis expansion analysis with exogenous variables: Forecasting electricity prices with NBEATSx. arXiv. https://arxiv.org/abs/2104.05522v6
- Pinto, A.C.V., Fernandes, T.E., De Lima E Silva, P.C.L., Guimarães, F.G., Wagner, C., & De Aguiar, E. P. (2022). Interval type-2 fuzzy set based time series forecasting using a data-driven partitioning approach. Evolving Systems, 13, 703–721. https://doi.org/10.1007/s12530-022-09452-2
- Rangapuram, S. S., Werner, L., Benidis, K., Mercado, P., Gasthaus, J., & Januschowski, T. (2021). End-to-End Learning of Coherent Probabilistic Forecasts for Hierarchical Time Series. In M. Meila, & T. Zhang (Eds.), Proceedings of Machine Learning Research: Vol. 139. Proceedings of the 38th International Conference on Machine Learning (pp. 8832–8843). PMLR. http://proceedings.mlr.press/v139/rangapuram21a/rangapuram21a.pdf
- Rasul, K., Seward, C., Schuster, I., & Vollgraf, R. (2021). Autoregressive Denoising Diffusion Models for Multivariate Probabilistic Time Series Forecasting. In M. Meila, & T. Zhang (Eds.), Proceedings of Machine Learning Research: Vol. 139. Proceedings of the 38th International Conference on Machine Learning (pp. 8857–8868). PMLR. http://proceedings.mlr.press/v139/rasul21a/rasul21a.pdf
- Sadaei, H. J., & Lee, M. H. (2014). Multilayer Stock Forecasting Model Using Fuzzy Time Series. The Scientific World Journal, 2014, 1–10. https://doi.org/10.1155/2014/610594
- Shah, M. (2012). Fuzzy based trend mapping and forecasting for time series data. Expert Systems with Applications, 39(7), 6351-6358. https://doi.org/10.1016/j.eswa.2011.12.036
- Singh, P., & Borah, B. (2013). High-order fuzzy-neuro expert system for time series forecasting. Knowledge Based Systems, 46, 12–21. https://doi.org/10.1016/j.knosys.2013.01.030
- Soloviev, V., Bielinskyi, A., & Solovieva, V. (2019). Entropy analysis of crisis phenomena for DJIA index. CEUR Workshop Proceedings, 2393, 434-449. http://ceur-ws.org/Vol-2393/paper_375.pdf
- Soloviev, V., Bielinskyi, A., Serdyuk, O., Solovieva, V., & Semerikov, S. (2020). Lyapunov exponents as indicators of the stock market crashes. CEUR Workshop Proceedings, 2732, 455-470. http://ceur-ws.org/Vol-2732/20200455.pdf
- Soloviev, V., Solovieva, V., & Tuliakova, A. (2019). Visibility graphs and precursors of stock crashes. Neuro-Fuzzy Modeling Techniques in Economics, 8, 3-29. http://doi.org/10.33111/nfmte.2019.003
- Song, Q., & Chissom, B. S. (1993). Forecasting enrollments with fuzzy time series — Part I. Fuzzy Sets and Systems, 54(1), 1–9. https://doi.org/10.1016/0165-0114(93)90355-l
- Song, Q., & Chissom, B. S. (1993). Fuzzy time series and its models. Fuzzy Sets and Systems, 54(3), 269–277. https://doi.org/10.1016/0165-0114(93)90372-o
- Song, Q., & Chissom, B. S. (1994). Forecasting enrollments with fuzzy time series — part II. Fuzzy Sets and Systems, 62(1), 1–8. https://doi.org/10.1016/0165-0114(94)90067-1
- Sun, B., Guo, H., Karimi, H. R., Ge, Y., & Xiong, S. (2015). Prediction of stock index futures prices based on fuzzy sets and multivariate fuzzy time series. Neurocomputing, 151(3), 1528–1536. https://doi.org/10.1016/j.neucom.2014.09.018
- Woo, G., Liu, C., Sahoo, D., Kumar, A., & Hoi, S. (2022). DeepTIMe: Deep Time-Index Meta-Learning for Non-Stationary Time-Series Forecasting. arXiv. https://arxiv.org/abs/2207.06046
- Wu, H., Xu, J., Wang, J., & Long, M. (2021). Autoformer: Decomposition transformers with auto-correlation for long-term series forecasting. In M. Ranzato, A. Beygelzimer, Y. Dauphin, P.S. Liang, & J. Wortman Vaughan (Eds.), Advances in Neural Information Processing Systems: Vol. 34 (pp. 22419-22430). Curran Associates, Inc. https://proceedings.neurips.cc/paper_files/paper/2021/file/bcc0d400288793e8bdcd7c19a8ac0c2b-Paper.pdf
- Yahoo. (2022). Yahoo! Finance [Data set]. Retrieved September 15, 2022, from https://www.finance.yahoo.com
- Yolcu, O. C., Yolcu, U., Egrioglu, E., & Aladag, C. H. (2016). High order fuzzy time series forecasting method based on an intersection operation. Applied Mathematical Modelling, 40(19–20), 8750–8765. https://doi.org/10.1016/j.apm.2016.05.012
- Yu, T. H. (2005). Weighted fuzzy time series models for TAIEX forecasting. Physica A: Statistical Mechanics and Its Applications, 349(3–4), 609–624. https://doi.org/10.1016/j.physa.2004.11.006
- Yu, T. H.-K., & Huarng, K.-H. (2008). A bivariate fuzzy time series model to forecast the TAIEX. Expert Systems with Applications, 34(4), 2945–2952. https://doi.org/10.1016/j.eswa.2007.05.016
- Zadeh, L. A. (1965). Fuzzy sets. Information and Control, 8(3), 338–353. https://doi.org/10.1016/s0019-9958(65)90241-x
- Zadeh, L. A. (1975). The concept of a linguistic variable and its application to approximate reasoning—I. Information Sciences, 8(3), 199–249. https://doi.org/10.1016/0020-0255(75)90036-5
- Zhou, H., Zhang, S., Peng, J., Zhang, S., Li, J., Xiong, H., & Zhang, W. (2021). Informer: Beyond efficient transformer for long sequence time-series forecasting. In Proceedings of the AAAI conference on artificial intelligence: Vol. 35(12) (pp. 11106-11115). AAAI Press. https://doi.org/10.1609/aaai.v35i12.17325