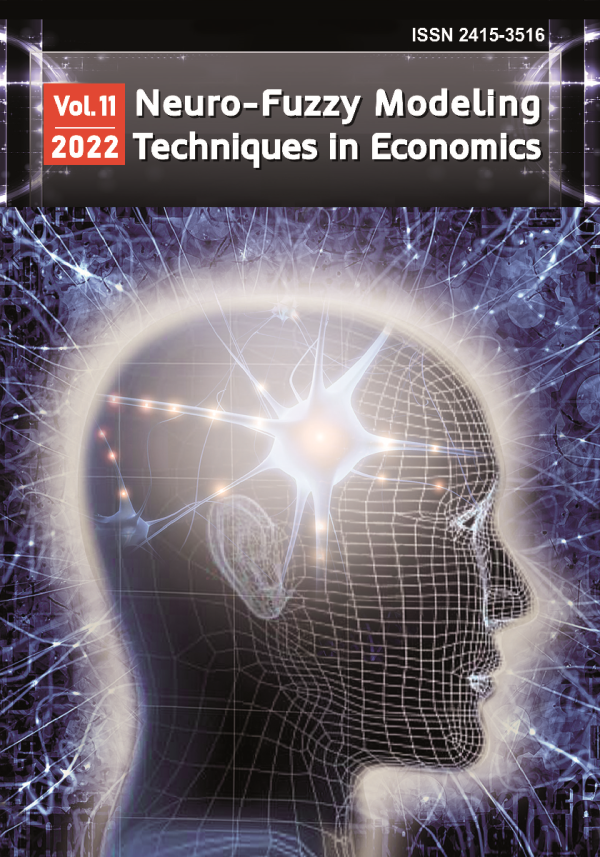
Neuro-Fuzzy Modeling Techniques in Economics
ISSN 2415-3516
Comparative analysis of the effectiveness of dimensionality reduction algorithms and clustering methods on the problem of modelling economic growth
DOI:
10.33111/nfmte.2023.067
Анотація:
Abstract: This article is devoted to the research of economic growth of countries by identifying patterns in historical data sets on macroeconomic indicators. Using machine learning techniques, namely cluster analysis methodology in combination with data transformation algorithms, in particular dimensionality reduction, groups of countries with similar patterns in the structure of the economy, availability of production factors, internal and external economic activity and development dynamics were formed. The novelty of the article is the approach to selecting optimal clustering and dimensionality reduction algorithms by quantifying the results of their work. The evaluation of the dimensionality reduction methods was carried out using the cumulative variance indicator, and the clustering methods were assessed based on the aggregate indicator proposed in the article, which combines the standardized Davies-Bouldin, Calinski-Harabasz indices and the Silhouette coefficient. According to calculations, among the 11 considered methods of dimensionality reduction, the most effective is the Kernel PCA algorithm, while among the 7 clustering methods, K-means is the most effective for this task with a given set of indicators. The study was conducted on 6 five-year time intervals from 1991 to 2020 with a focus on the Ukrainian economy. According to the research, Ukraine’s economy migrated from the “post-Soviet” cluster (first half of the 1990s) to the Eastern European cluster (second half of the 2010s) over the period under consideration, which indicates real economic growth and gradual integration with the European Union.
Ключові слова:
Key words: economic growth, cluster analysis, dimensionality reduction, machine learning
УДК:
UDC:
JEL: C38 F43 O41
To cite paper
In APA style
Poznyak, S., & Kolyada, Y. (2023). Comparative analysis of the effectiveness of dimensionality reduction algorithms and clustering methods on the problem of modelling economic growth. Neuro-Fuzzy Modeling Techniques in Economics, 12, 67-110. http://doi.org/10.33111/nfmte.2023.067
In MON style
Позняк С., Коляда Ю.В. Comparative analysis of the effectiveness of dimensionality reduction algorithms and clustering methods on the problem of modelling economic growth. Нейро-нечіткі технології моделювання в економіці. 2023. № 12. С. 67-110. http://doi.org/10.33111/nfmte.2023.067 (дата звернення: 16.09.2024).
With transliteration
Poznyak, S., Kolyada, Y. (2023) Comparative analysis of the effectiveness of dimensionality reduction algorithms and clustering methods on the problem of modelling economic growth. Neuro-Fuzzy Modeling Techniques in Economics, no. 12. pp. 67-110. http://doi.org/10.33111/nfmte.2023.067 (accessed 16 Sep 2024).
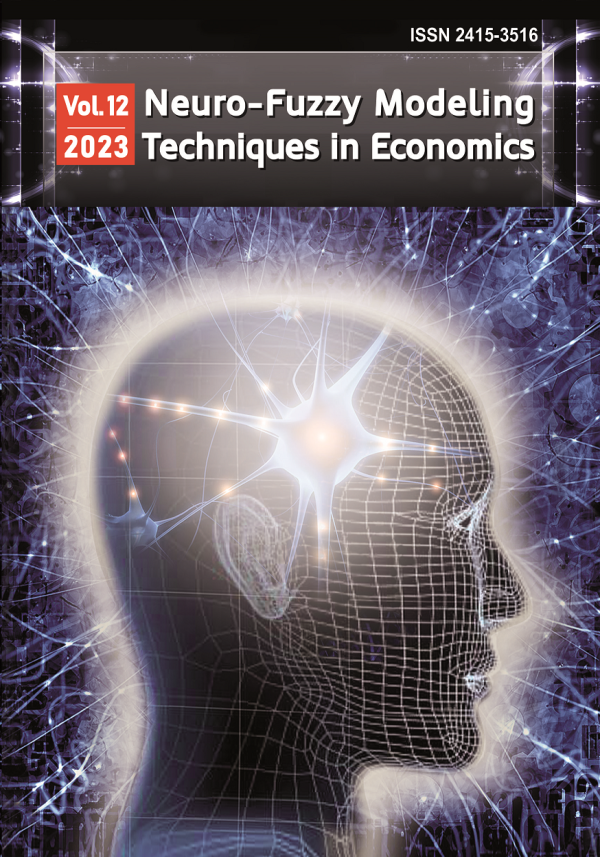
Download Paper
67
Views
27
Downloads
0
Cited by
- Solow, R. M. (1956). A Contribution to the Theory of Economic Growth. The Quarterly Journal of Economics, 70(1), 65-94. https://doi.org/10.2307/1884513
- Solow, R. M. (1957). Technical Change and the Aggregate Production Function. The Review of Economics and Statistics, 39(3), 312-320. https://doi.org/10.2307/1926047
- Swan, T. W. (1956). Economic Growth and Capital Accumulation. Economic Record, 32(2), 334-361. https://doi.org/10.1111/j.1475-4932.1956.tb00434.x
- Ramsey, F. P. (1928). A mathematical theory of saving. The Economic Journal, 38(152), 543-559. https://doi.org/10.2307/2224098
- Cass, D. (1965). Optimum Growth in an Aggregative Model of Capital Accumulation. The Review of Economic Studies, 32(3), 233-240. https://doi.org/10.2307/2295827
- Koopmans, T.C. (1963). On the Concept of Optimal Economic Growth. Cowles Foundation Discussion Papers, Article 163. https://elischolar.library.yale.edu/cowles-discussion-paper-series/392
- Uzawa, H. (1965). Optimal Technical Change in an Aggregative Model of Economic Growth. International Economic Review, 6(1), 18-31. https://doi.org/10.2307/2525621
- Lucas, R. E. (1988). On the Mechanics of Economic Development. Journal of Monetary Economics, 22(1), 3-42. https://doi.org/10.1016/0304-3932(88)90168-7
- Mankiw, N. G., Romer, D., & Weil, D. N. (1992). A Contribution to the Empirics of Economic Growth. The Quarterly Journal of Economics, 107(2), 407-437. https://doi.org/10.2307/2118477
- Romer, P. M. (1989). Human Capital and Growth: Theory and Evidence (Working Paper No. 3173). National Bureau of Economic Research. https://doi.org/10.3386/w3173
- Romer, P. M. (1989). Endogenous Technological Change (Working Paper No. 3210). National Bureau of Economic Research. https://doi.org/10.3386/w3210
- Kitchin, J. (1923). Cycles and Trends in Economic Factors. The Review of Economics and Statistics, 5(1), 10-16. https://doi.org/10.2307/1927031
- Kuznets, S. (1960). Economic Growth Of Small Nations. In E.A.G. Robinson (Ed.), International Economic Association Series. Economic Consequences of the Size of Nations (pp. 14-32). Palgrave Macmillan. https://doi.org/10.1007/978-1-349-15210-0_2
- Korotayev, A. V., & Tsirel, S. V. (2010). A Spectral Analysis of World GDP Dynamics: Kondratieff Waves, Kuznets Swings, Juglar and Kitchin Cycles in Global Economic Development, and the 2008-2009 Economic Crisis. Structure and Dynamics, 4(1), Article 3306. https://doi.org/10.5070/sd941003306
- Strelchenko, I. (2019). Modeling of cross-border spreading of financial crisis. Neuro-Fuzzy Modeling Techniques in Economics, 8, 147-174. http://doi.org/10.33111/nfmte.2019.147
- Matviychuk, A., Strelchenko, I., Vashchaiev, S., & Velykoivanenko, H. (2019). Simulation of the Crisis Contagion Process Between Countries with Different Levels of Socio-Economic Development. CEUR Workshop Proceedings, 2393(II), 485-496. http://ceur-ws.org/Vol-2393/paper_423.pdf
- Lukianenko, D., & Strelchenko, I. (2021). Neuromodeling of features of crisis contagion on financial markets between countries with different levels of economic development. Neuro-Fuzzy Modeling Techniques in Economics, 10, 136-163. http://doi.org/10.33111/nfmte.2021.136
- Kriegel, H.-P., Schubert, E., & Zimek, A. (2017). The (black) art of runtime evaluation: Are we comparing algorithms or implementations? Knowledge and Information Systems, 52(2), 341-378. https://doi.org/10.1007/s10115-016-1004-2
- Zare, H., Shooshtari, P., Gupta, A., & Brinkman, R. R. (2010). Data reduction for spectral clustering to analyze high throughput flow cytometry data. BMC Bioinformatics, 11, Article 403. https://doi.org/10.1186/1471-2105-11-403
- Ward, J. H. (1963). Hierarchical Grouping to Optimize an Objective Function, Journal of the American Statistical Association, 58(301), 236-244. https://doi.org/10.1080/01621459.1963.10500845
- Zhang, T., Ramakrishnan, R., & Livny, M. (1996). BIRCH: an efficient data clustering method for very large databases. ACM SIGMOD Record, 25(2), 103-114. https://doi.org/10.1145/233269.233324
- Nielsen, F. (2016). Hierarchical Clustering. In Undergraduate Topics in Computer Science. Introduction to HPC with MPI for Data Science (pp. 195-211). Springer. https://doi.org/10.1007/978-3-319-21903-5_8
- Reynolds, D. A., & Rose, R. C. (1995). Robust text-independent speaker identification using Gaussian mixture speaker models. IEEE Transactions on Speech and Audio Processing, 3(1), 72-83. https://doi.org/10.1109/89.365379
- Frey, B. J., & Dueck, D. (2007). Clustering by Passing Messages Between Data Points. Science, 315(5814), 972-976. https://doi.org/10.1126/science.1136800
- Cheng, Y. (1995). Mean shift, mode seeking, and clustering. IEEE Transactions on Pattern Analysis and Machine Intelligence, 17(8), 790-799. https://doi.org/10.1109/34.400568
- Ester, M., Kriegel, H.-P., Sander, J., & Xu, X. (1996). A density-based algorithm for discovering clusters in large spatial databases with noise. In Proceedings of the Second International Conference on Knowledge Discovery and Data Mining (pp. 226-231). AAAI. https://aaai.org/papers/kdd96-037-a-density-based-algorithm-for-discovering-clusters-in-large-spatial-databases-with-noise/
- Campello, R. J. G. B., Moulavi, D., Zimek, A., & Sander, J. (2015). Hierarchical Density Estimates for Data Clustering, Visualization, and Outlier Detection. ACM Transactions on Knowledge Discovery from Data, 10(1), Article 5. https://doi.org/10.1145/2733381
- Ankerst, M., Breunig, M., Kriegel, H.-P., & Sander, J. (1999). OPTICS: ordering points to identify the clustering structure. ACM SIGMOD Record, 28(2), 49-60. http://dx.doi.org/10.1145/304181.304187
- Deltuvaitė, V., & Sinevičienė, L. (2014). Investigation of Relationship between Financial and Economic Development in the EU Countries. Procedia Economics and Finance, 14, 173-180. https://doi.org/10.1016/s2212-5671(14)00700-x
- Enzmann, P., & Moesli, M. (2022). Seizing opportunities: ASEAN country cluster readiness in light of the fourth industrial revolution. Asia and the Global Economy, 2(1), Article 100021. https://doi.org/10.1016/j.aglobe.2021.100021
- Koutsoukis, N.-S. (2015). Global Political Economy Clusters: The World as Perceived through Black-box Data Analysis of Proxy Country Rankings and Indicators. Procedia Economics and Finance, 33, 18-45. https://doi.org/10.1016/s2212-5671(15)01691-3
- Peruzzi, M., & Terzi, A. (2021). Accelerating Economic Growth: The Science beneath the Art. Economic Modelling, 103, Article 105593. https://doi.org/10.1016/j.econmod.2021.105593
- Čadil, J., Petkovová, L., & Blatná, D. (2014). Human Capital, Economic Structure and Growth. Procedia Economics and Finance, 12, 85-92. https://doi.org/10.1016/s2212-5671(14)00323-2
- Cerqueti, R., & Ficcadenti, V. (2022). Combining rank-size and k-means for clustering countries over the COVID-19 new deaths per million. Chaos, Solitons & Fractals, 158, Article 111975. https://doi.org/10.1016/j.chaos.2022.111975
- Wulandari, L., & Yogantara, B. O. (2022). Algorithm Analysis of K-Means and Fuzzy C-Means for Clustering Countries Based on Economy and Health. Faktor Exacta, 15(2), 109-116. https://doi.org/10.30998/faktorexacta.v15i2.12106
- Jolliffe, I. (2002). Principal Component Analysis (2nd ed.). Springer New York. https://doi.org/10.1007/b98835
- DeAngelis, G. C., Ohzawa, I., & Freeman, R. D. (1995). Receptive-field dynamics in the central visual pathways. Trends in Neurosciences, 18(10), 451-458. https://doi.org/10.1016/0166-2236(95)94496-r
- Bingham, E., & Mannila, H. (2001). Random projection in dimensionality reduction: applications to image and text data. In Proceedings of the seventh ACM SIGKDD International Conference on Knowledge Discovery and Data Mining (KDD ’01) (pp. 245-250). Association for Computing Machinery. https://doi.org/10.1145/502512.502546
- Hyvärinen, A. (2013). Independent component analysis: recent advances. Philosophical Transactions of the Royal Society A: Mathematical, Physical and Engineering Sciences, 371(1984), Article 20110534. https://doi.org/10.1098/rsta.2011.0534
- Tenenbaum, J. B., de Silva, V., & Langford, J. C. (2000). A Global Geometric Framework for Nonlinear Dimensionality Reduction. Science, 290(5500), 2319-2323. https://doi.org/10.1126/science.290.5500.2319
- Mead, A. (1992). Review of the Development of Multidimensional Scaling Methods. Journal of the Royal Statistical Society. Series D (The Statistician), 41(1), 27-39. https://doi.org/10.2307/2348634
- Roweis, S. T., & Saul, L. K. (2000). Nonlinear Dimensionality Reduction by Locally Linear Embedding. Science, 290(5500), 2323-2326. https://doi.org/10.1126/science.290.5500.2323
- Linderman, G. C., & Steinerberger, S. (2017). Clustering with t-SNE, provably. arXiv. https://doi.org/10.48550/ARXIV.1706.02582
- Davies, D. L., & Bouldin, D. W. (1979). A Cluster Separation Measure. IEEE Transactions on Pattern Analysis and Machine Intelligence, PAMI-1(2), 224-227. https://doi.org/10.1109/tpami.1979.4766909
- Calinski, T., & Harabasz, J. (1974). A dendrite method for cluster analysis. Communications in Statistics, 3(1), 1-27. https://doi.org/10.1080/03610927408827101
- Rousseeuw, P. J. (1987). Silhouettes: A graphical aid to the interpretation and validation of cluster analysis. Journal of Computational and Applied Mathematics, 20, 53-65. https://doi.org/10.1016/0377-0427(87)90125-7
- Rahman, M. M., & Alam, K. (2021). Exploring the driving factors of economic growth in the world’s largest economies. Heliyon, 7(5), Article E07109. https://doi.org/10.1016/j.heliyon.2021.e07109
- The World Bank. (2023). World Development Indicators [Data set]. Retrieved February 1, 2023, from https://databank.worldbank.org/source/world-development-indicators
- Zhylinska, O., Bazhenova, O., Zatonatska, T., Dluhopolskyi, O., Bedianashvili, G., & Chornodid, I. (2020). Innovation processes and economic growth in the context of European integration. Scientific Papers of the University of Pardubice, Series D: Faculty of Economics and Administration, 28(3), Article 1209. https://doi.org/10.46585/sp28031209
- Scikit-learn. (n.d.). 2.3. Clustering. Retrieved February 20, 2023, from https://scikit-learn.org/stable/modules/clustering.html