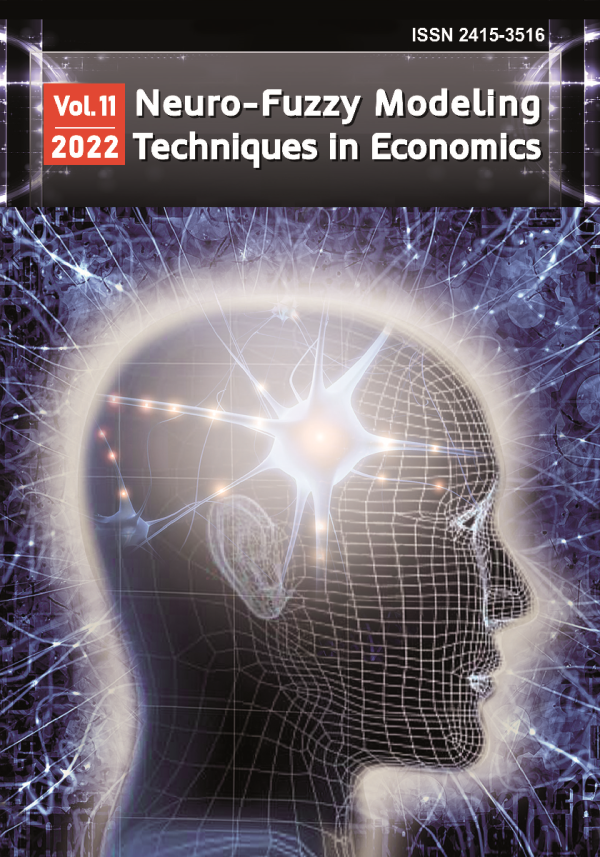
Neuro-Fuzzy Modeling Techniques in Economics
ISSN 2415-3516
Management of pharmaceutical online retail through a regional marketplace with neural network and statistical analytical tools
DOI:
10.33111/nfmte.2023.155
Анотація:
Abstract: The study is devoted to applying neural networks and statistical analytical tools for the estimation of efficiency of pharmaceutical retail. The empirical basis was data derived from the Ukrainian regional online marketplace. The article proposes and tests approach to analytical decision-making in online retail, which involves combining several clustering and forecasting models and methods, complementing each other accordingly. Analysis of the results of clustering and statistical processing of empirical datasets characterizing customer orders for goods in pharmacies, as well as the order-placement procedure, made it possible to identify patterns in the behaviour of customers and pharmacy staff in the process of fulfilling orders. We also created a perceptron-type neural network to predict the value of sold products as the final result of each commercial operation in a pharma store. The modelling results form the necessary conditions for determining management indicators and assessing the effectiveness of interactions with customers, which have practical value and potentially allow increasing sales and customer service levels.
Ключові слова:
Key words: online retail, pharmacy, consumers’ behaviour, Kohonen mapping, perceptron-type neural network, clustering, forecasting, commercial performance
УДК:
UDC:
JEL: C15 C38 C45 L81 M21 M31
To cite paper
In APA style
Oleksiuk, O., & Shafalyuk, O. (2023). Management of pharmaceutical online retail through a regional marketplace with neural network and statistical analytical tools. Neuro-Fuzzy Modeling Techniques in Economics, 12, 155-174. http://doi.org/10.33111/nfmte.2023.155
In MON style
Олексюк О.І., Шафалюк О.К. Management of pharmaceutical online retail through a regional marketplace with neural network and statistical analytical tools. Нейро-нечіткі технології моделювання в економіці. 2023. № 12. С. 155-174. http://doi.org/10.33111/nfmte.2023.155 (дата звернення: 12.07.2025).
With transliteration
Oleksiuk, O., Shafalyuk, O. (2023) Management of pharmaceutical online retail through a regional marketplace with neural network and statistical analytical tools. Neuro-Fuzzy Modeling Techniques in Economics, no. 12. pp. 155-174. http://doi.org/10.33111/nfmte.2023.155 (accessed 12 Jul 2025).
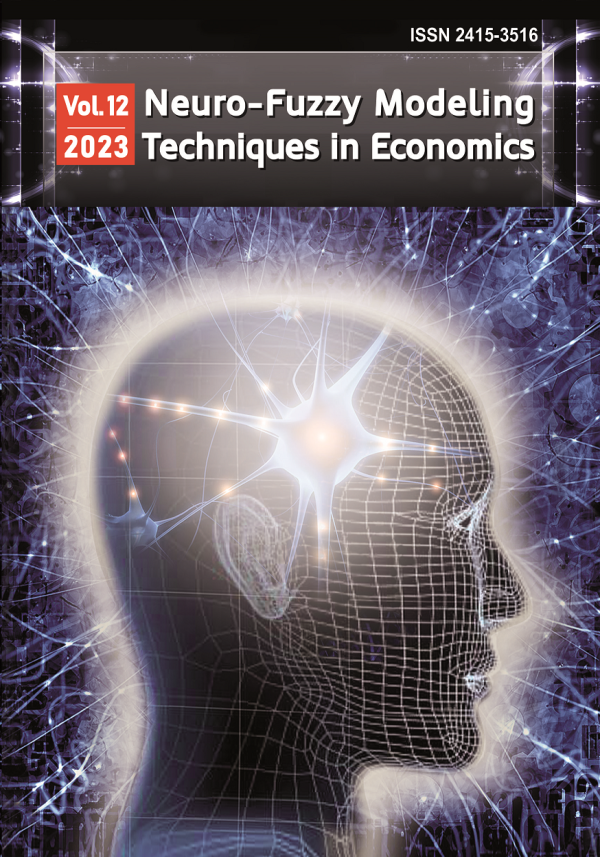
Download Paper
433
Views
76
Downloads
0
Cited by
- Zhang, X., Edwards, J., & Harding, J. (2007). Personalised online sales using web usage data mining. Computers in Industry, 58(8–9), 772-782. https://doi.org/10.1016/j.compind.2007.02.004
- Bradlow, E. T., Gangwar, M., Kopalle, P., & Voleti, S. (2017). The Role of Big Data and Predictive Analytics in Retailing. Journal of Retailing, 93(1), 79-95. https://doi.org/10.1016/j.jretai.2016.12.004
- Sulthana, A. R., & Ramasamy, S. (2019). Ontology and context based recommendation system using Neuro-Fuzzy Classification. Computers & Electrical Engineering, 74, 498-510. https://doi.org/10.1016/j.compeleceng.2018.01.034
- Nilashi, M., Abumalloh, R., Samad, S., Minaei-Bidgoli, B., Thi, H., Alghamdi, O., Ismail, M., & Ahmadi, H. (2023). The impact of multi-criteria ratings in social networking sites on the performance of online recommendation agents. Telematics and Informatics, 76, Article 101919. https://doi.org/10.1016/j.tele.2022.101919
- Liao, Sh.-H., Chen, Ch.-M., & Wu, Ch.-H. (2008). Mining customer knowledge for product line and brand extension in retailing. Expert Systems with Applications, 34(3), 1763-1776. https://doi.org/10.1016/j.eswa.2007.01.036
- Voronenko, I., Nehrey, M., Kostenko, S., Lashchyk, I., & Niziaieva, V. (2021). Advertising Strategy Management in Internet Marketing. Journal of Information Technology Management, 13(Special Issue: Advanced Innovation Topics in Business and Management), 35-47. https://doi.org/10.22059/jitm.2021.82603
- Kopytko, M., Boychuk, I., Balyk, U., Mykhailyk, N., & Hryhoruk, P. (2023). Formation of Marketing Strategy for Sustainable Development of Enterprises in the Domestic Market. Review of Economics and Finance, 21, 2271-2278. https://doi.org/10.55365/1923.x2023.21.243
- Balan, V. (2022). Enterprise strategies stratification based on the fuzzy matrix approach. Neuro-Fuzzy Modeling Techniques in Economics, 11, 124-156. http://doi.org/10.33111/nfmte.2022.124
- Kokoç, M., Ersöz, S., & Aktepe, A. (2020) A Fuzzy Inference System Proposal for Selecting Marketing Strategy. International Journal of Eastern Anatolia Science Engineering and Design, 2(1), 1-21. https://dergipark.org.tr/en/pub/ijeased/issue/50819/653763
- Leung, K.H., Choy, K.L., Ho, G.T.S., Lee Carman, K.M., Lam, H.Y., & Luk, C.C. (2019). Prediction of B2C e-commerce order arrival using hybrid autoregressive-adaptive neuro-fuzzy inference system (AR-ANFIS) for managing fluctuation of throughput in e-fulfilment centres. Expert Systems with Applications, 134, 304-324. https://doi.org/10.1016/j.eswa.2019.05.027
- Malhotra, M. K., Sharma, S., & Nair, S. S. (1999). Decision making using multiple models. European Journal of Operational Research, 114(1), 1-14. https://doi.org/10.1016/S0377-2217(98)00037-X
- Rodrigues, S. E., & Serra, G. L.O. (2022). An approach for evolving neuro-fuzzy forecasting of time series based on parallel recursive singular spectrum analysis. Fuzzy Sets and Systems, 443(B), 1-29. https://doi.org/10.1016/j.fss.2021.09.009
- Wang, Ch. (2022). Efficient customer segmentation in digital marketing using deep learning with swarm intelligence approach. Information Processing & Management, 59(6), Article 103085. https://doi.org/10.1016/j.ipm.2022.103085
- Kuo, R.J., An, Y.L., Wang, H.S., & Chung, W.J. (2006). Integration of self-organizing feature maps neural network and genetic K-means algorithm for market segmentation. Expert Systems with Applications, 30(2), 313-324. https://doi.org/10.1016/j.eswa.2005.07.036
- Mazur, H., Burkina, N., Popovskyi, Y., Vasylenko, N., Zaiachkovskyi, V., Lavrov, R., & Kozlovskyi, S. (2023). Customer Classification and Decision Making in the Digital Economy based on Scoring Models. WSEAS Transactions on Business and Economics, 20, 800-814. https://doi.org/10.37394/23207.2023.20.74
- Savych, O., Molchanova, E., Fedorchenko, A., & Kovtoniuk, K. (2021). Modeling of Key Marketing Parameters of Development of the EU Car Market and its Clusterization. In Proceedings of 2021 11th International Conference on Advanced Computer Information Technologies (ACIT) (pp. 364-367). IEEE. https://doi.org/10.1109/ACIT52158.2021.9548474
- France, S. L., & Ghose, S. (2019). Marketing analytics: Methods, practice, implementation, and links to other fields. Expert Systems with Applications, 119, 456-475. https://doi.org/10.1016/j.eswa.2018.11.002
- Turlakova, S. (2022). Modeling the values of reflexive characteristics of agents within the management of herd behavior at the enterprises. Neuro-Fuzzy Modeling Techniques in Economics, 11, 48-77. http://doi.org/10.33111/nfmte.2022.048
- Lukianenko, D., Matviychuk, A., Lukianenko, L., & Dvornyk, I. (2023). University competitiveness in the knowledge economy: a Kohonen map approach. CEUR Workshop Proceedings, 3465, 236–250. https://ceur-ws.org/Vol-3465/paper23.pdf
- Velykoivanenko, H., Korchynskyi, V., & Chernyshova, V. (2016). Doslidzhennia efektu perenavchannia neironnykh merezh na prykladi zadachi aplikatsiinoho skorynhu [Study of the neural networks overfitting effect on the example of the problem of application scoring]. Neiro-Nechitki Tekhnolohii Modelyuvannya v Ekonomitsi (Neuro-Fuzzy Modeling Techniques in Economics), 5, 3-23. http://doi.org/10.33111/nfmte.2016.003 [in Ukrainian]
- Sharma, A., Randhawa, P., & Alharbi, H. F. (2022). Statistical and Machine Learning Approaches to Predict the Next Purchase Date: A Review. In Proceedings of 2022 4th International Conference on Applied Automation and Industrial Diagnostics (ICAAID) (pp. 1-7). IEEE. http://doi.org/10.1109/ICAAID51067.2022.9799494