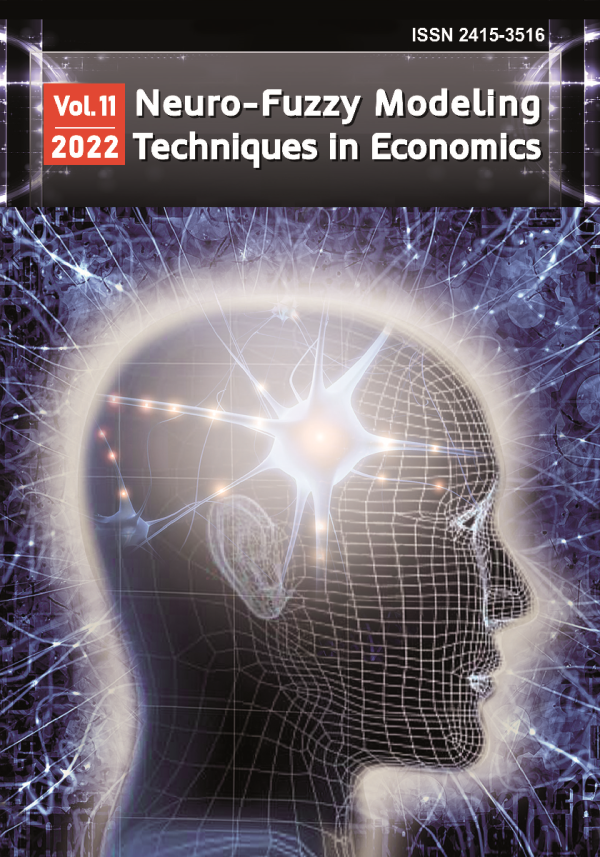
Neuro-Fuzzy Modeling Techniques in Economics
ISSN 2415-3516
Identifying moments of decision making on trade in financial time series using fuzzy cluster analysis
DOI:
10.33111/nfmte.2023.175
Анотація:
Abstract: The article investigates the problem of identifying trading decision points in financial time series using the Fuzzy C-Means (FCM) method. The authors argue that classical forecasting methods have limited effectiveness for decision-making in trading, as they do not take into account market structure and nonlinear patterns. The proposed methodology involves analysing time series using additional features derived from technical indicators (MACD, Stochastic) and further clustering based on FCM, which allows identifying market entry and exit points. In contrast to traditional approaches based on the assessment of forecasting accuracy (e.g. MAE, RMSE, MAPE), this study focuses on financially oriented metrics such as Net Profit, Max Drawdown, Win Rate and Profit Factor, which more accurately reflect the effectiveness of trading strategies in real market conditions. Experiments on the currency pairs EUR/USD, AUD/USD, USD/JPY, USD/CAD on daily and four-hour timeframes have demonstrated that the use of the proposed approach can improve the efficiency of trading strategies. The simulation results showed fairly high stable profitability results with low risks (drawdown). The proposed approach can be useful in developing automated trading systems and further research in the field of financial analytics.
Ключові слова:
Key words: financial time series, trading, cluster analysis, fuzzy c-means, technical analysis, financial performance metrics, trend prediction
УДК:
UDC:
JEL: C38 C53 C63 G10
To cite paper
In APA style
Kabachii, V., Maslii, R., Kozlovskyi, S., & Dronchack, O. (2023). Identifying moments of decision making on trade in financial time series using fuzzy cluster analysis. Neuro-Fuzzy Modeling Techniques in Economics, 12, 175-205. http://doi.org/10.33111/nfmte.2023.175
In MON style
Kabachii V., Maslii R., Kozlovskyi S., Dronchack O. Identifying moments of decision making on trade in financial time series using fuzzy cluster analysis. Нейро-нечіткі технології моделювання в економіці. 2023. № 12. С. 175-205. http://doi.org/10.33111/nfmte.2023.175 (дата звернення: 15.07.2025).
With transliteration
Kabachii, V., Maslii, R., Kozlovskyi, S., Dronchack, O. (2023) Identifying moments of decision making on trade in financial time series using fuzzy cluster analysis. Neuro-Fuzzy Modeling Techniques in Economics, no. 12. pp. 175-205. http://doi.org/10.33111/nfmte.2023.175 (accessed 15 Jul 2025).
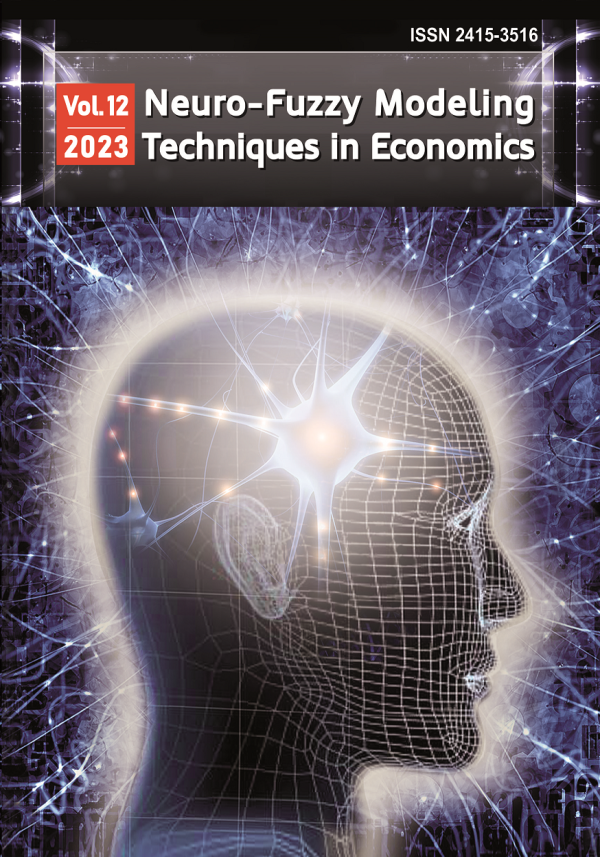
Download Paper
320
Views
57
Downloads
0
Cited by
- Tsay, R. S. (2005). Analysis of financial time series (2nd ed.). John Wiley & Sons, Inc,
- Brockwel, P. J., Davis, R. A. (2016). Introduction to Time Series and Forecasting (3rd ed.). Springer Cham. https://doi.org/10.1007/978-3-319-29854-2
- Matviychuk, A. (2006). Fuzzy logic approach to identification and forecasting of financial time series using Elliott wave theory. Fuzzy economic review, 11(2), 51-68. https://doi.org/10.25102/fer.2006.02.04
- Achelis, S. B. (2013). Technical Analysis from A to Z (2nd ed.). McGraw Hill.
- Elder, A. (1993). Trading for a Living: Psychology, Trading Tactics, Money Management. Wiley.
- Kuchansky, A., Nikolenko, V., & Rachenko, A. (2015). A method for identifying trends in financial time series based on trend models of forecasting. Management of Development of Complex Systems, 24, 84-89. https://urss.knuba.edu.ua/ua/zbirnyk-24/article-862 [in Ukrainian]
- Wilson, S. J. (2016). Trend Detection and Pattern Recognition in Financial Time Series [Doctoral dissertation, George Mason University]. MARS. https://doi.org/10.13021/MARS/7317
- Bakai, Ye. І., Kabachii, V. V., & Maslii, R. V. (2017). Decision-making Model on Financial Time Series Based on a Couple of Moving Averages Using the Assessment of Different Timeframes. Visnyk of Vinnytsia Polytechnical Institute, 1, 70-77. https://visnyk.vntu.edu.ua/index.php/visnyk/article/view/2014 [in Ukrainian]
- Dash, T., Chitlangia, S., Ahuja, A., & Srinivasan, A. (2021). Incorporating domain knowledge into deep neural networks. arXiv. https://doi.org/10.48550/arXiv.2103.00180
- Borghesi, A., Baldo, F., & Milano, M. (2020). Improving Deep Learning Models via Constraint-Based Domain Knowledge: A Brief Survey. arXiv. https://doi.org/10.48550/arXiv.2005.10691
- Kozlovskyi, S., Mazur, H., Vdovenko, N., Shepel, T., & Kozlovskyi, V. (2018). Modeling and forecasting the level of state stimulation of agricultural production in Ukraine based on the theory of fuzzy logic. Montenegrin Journal of Economics, 14(3), 37-53. https://doi.org/10.14254/1800-5845/2018.14-3.3
- Bielinskyi, A., Soloviev, V., Solovieva, V., & Velykoivanenko, H. (2022). Fuzzy time series forecasting using semantic artificial intelligence tools. Neuro-Fuzzy Modeling Techniques in Economics, 11, 157-198. http://doi.org/10.33111/nfmte.2022.157
- Nair, B. B., Kumar, P. S., Sakthivel, N. R., & Vipin, U. (2017). Clustering stock price time series data to generate stock trading recommendations: An empirical study. Expert Systems with Applications, 70, 20-36. https://doi.org/10.1016/j.eswa.2016.11.002
- Kaminskyi, A., Miroshnychenko, I., & Pysanets, K. (2019). Risk and return for cryptocurrencies as alternative investment: Kohonen maps clustering. Neuro-Fuzzy Modeling Techniques in Economics, 8, 175-193. http://doi.org/10.33111/nfmte.2019.175
- Kononova, K., & Dek, A. (2018). Investigation of traders’ behavioral characteristics: experimental economics methods and machine learning technologies. Neuro-Fuzzy Modeling Techniques in Economics, 7, 148-167. http://doi.org/10.33111/nfmte.2018.148
- Matviychuk, A., Strelchenko, I., Vashchaiev, S., & Velykoivanenko, H. (2019). Simulation of the Crisis Contagion Process between Countries with Different Levels of Socio-Economic Development. CEUR Workshop Proceedings, 2393, 485-496. http://ceur-ws.org/Vol-2393/paper_423.pdf
- Enke, D., Grauer, M., & Mehdiyev, N. (2011). Stock market prediction with multiple regression, fuzzy type-2 clustering and neural networks. Procedia Computer Science, 6, 201-206. https://doi.org/10.1016/j.procs.2011.08.038
- Mehmanpazir, F., & Asadi, S. (2017). Development of an evolutionary fuzzy expert system for estimating future behavior of stock price. Journal of Industrial Engineering International, 13, 29-46. https://doi.org/10.1007/s40092-016-0165-7
- Shi, Y., Li, B., Du, G., & Dai, W. (2021). Clustering framework based on multi-scale analysis of intraday financial time series. Physica A: Statistical Mechanics and its Applications, 567, Article 125728. https://doi.org/10.1016/j.physa.2020.125728
- Bandara, K., Bergmeir, C., & Smyl, S. (2020). Forecasting across time series databases using recurrent neural networks on groups of similar series: A clustering approach. Expert systems with applications, 140, Article 112896. https://doi.org/10.1016/j.eswa.2019.112896
- Long, J., Chen, Z., He, W., Wu, T., & Ren, J. (2020). An integrated framework of deep learning and knowledge graph for prediction of stock price trend: An application in Chinese stock exchange market. Applied Soft Computing, 91, Article 106205. https://doi.org/10.1016/j.asoc.2020.106205
- Wang, X., Yang, K., & Liu, T. (2021). Stock Price Prediction Based on Morphological Similarity Clustering and Hierarchical Temporal Memory. IEEE Access, 9, 67241-67248. https://doi.org/10.1109/ACCESS.2021.3077004
- Ozer, F., & Okan Sakar, C. (2022). An automated cryptocurrency trading system based on the detection of unusual price movements with a time-series clustering-based approach. Expert Systems with Applications, 200, Article 117017. https://doi.org/10.1016/j.eswa.2022.117017
- Sáenz, J. V., Quiroga, F. M., & Bariviera, A. F. (2023). Data vs. information: Using clustering techniques to enhance stock returns forecasting. International Review of Financial Analysis, 88, Article 102657. https://doi.org/10.1016/j.irfa.2023.102657
- D’Urso, P., De Giovanni, L., & Massari, R. (2021). Trimmed fuzzy clustering of financial time series based on dynamic time warping. Annals of operations research, 299, 1379-1395. https://doi.org/10.1007/s10479-019-03284-1
- Hartigan, J.A., & Wong, M.A. (1979). A k-means clustering algorithm. Journal of the Royal Statistical Society. Series C, 28(1), 100-108. https://doi.org/10.2307/2346830
- Dunn, J. C. (1973). A Fuzzy Relative of the ISODATA Process and Its Use in Detecting Compact Well-Separated Clusters. Journal of Cybernetics, 3(3), 32–57. https://doi.org/10.1080/01969727308546046
- Goddard, J., de los Cobos Silva, S.G., & Gutiérrez Andrade, M.A. (2006). Comparison of k-means and fuzzy c-means using background knowledge. Fuzzy economic review, 11(2), 3-16. https://doi.org/10.25102/fer.2006.02.01
- Miyamoto, S., Ichihashi, H., & Honda, K. (2008). Algorithms for Fuzzy Clustering. Methods in c-Means Clustering with Applications. Springer. https://doi.org/10.1007/978-3-540-78737-2
- MetaTrader 4. (2023, April 5). MetaTrader 4 – the most popular Forex trading platform. https://www.metatrader4.com/en
- Murphy, J.J. (1999). Technical Analysis of the Financial Markets: A Comprehensive Guide to Trading Methods and Applications. NYIF.
- Haryono, A. T., Sarno, R., & Sungkono, K. R. (2023). Transformer-Gated Recurrent Unit Method for Predicting Stock Price Based on News Sentiments and Technical Indicators. IEEE Access, 11, 77132-77146. https://doi.org/10.1109/ACCESS.2023.3298445